How to Apply Trading AI Models to Predict Market Trends with 90% Accuracy
From Data to Decisions: Your Path to Trading Success
Discovering how to apply trading AI has transformed the landscape of financial markets, creating unprecedented opportunities for investors to achieve remarkable accuracy in market predictions.
The fusion of artificial intelligence and trading has revolutionized how traders approach market analysis, offering a scientific approach to what was once considered more art than science.
Traditional trading methods, relying solely on human intuition and basic technical analysis, often fall short in today’s complex market environment.
Modern traders who learn how to apply trading AI systems gain a significant competitive advantage, leveraging powerful algorithms that can process vast amounts of data in milliseconds.
This comprehensive guide will walk you through the essential steps of implementing AI in your trading strategy, ensuring you understand both the theoretical foundations and practical applications.
By the end of this article, you’ll have a clear roadmap for how to apply trading AI models effectively, potentially achieving prediction accuracies that seemed impossible just a few years ago.
We strongly recommend that you check out our guide on how to take advantage of AI in today’s passive income economy.
Table of Contents
Understanding the Foundations of AI Trading Models
Before diving into how to apply trading AI, it’s crucial to understand the basic building blocks that make these systems work.
Machine learning algorithms form the core of successful trading AI systems, particularly supervised learning models that can identify patterns in historical market data.
These algorithms excel at recognizing complex relationships between various market indicators that might be invisible to the human eye.
Neural networks, a sophisticated type of AI, can process multiple layers of market information simultaneously, making them particularly effective for price prediction.
Understanding the different types of AI models available is crucial when learning how to apply trading AI effectively in your trading strategy.
Types of AI Models for Trading
1. Time Series Models
ARIMA (Autoregressive Integrated Moving Average) models remain popular among traders learning how to apply trading AI for short-term predictions.
Long Short-Term Memory (LSTM) networks excel at capturing long-term dependencies in market data, making them ideal for trend prediction.
Prophet, developed by Facebook, offers robust capabilities for handling seasonal patterns and market anomalies.
These models form the foundation of how to apply trading AI in practical trading scenarios, each serving specific purposes in your overall strategy.
2. Sentiment Analysis Models
Natural Language Processing (NLP) models analyze news articles, social media posts, and financial reports to gauge market sentiment.
Traders who successfully learn how to apply trading AI for sentiment analysis gain valuable insights into market psychology.
These models can process thousands of news articles per second, providing real-time sentiment indicators that influence trading decisions.
Understanding how to apply trading AI in sentiment analysis can give traders a significant edge in predicting market movements based on public opinion.
Data Collection and Preparation
The success of any AI trading system depends heavily on the quality and quantity of data used for training.
Learning how to apply trading AI effectively begins with gathering high-quality historical market data from reliable sources.
This data should include price information, volume data, technical indicators, and fundamental market data.
Successful traders who know how to apply trading AI understand the importance of clean, well-structured data.
Data Cleaning and Preprocessing
Raw market data often contains noise, gaps, and inconsistencies that need to be addressed before training AI models.
Understanding how to apply trading AI preprocessing techniques is crucial for maintaining data quality.
Standard preprocessing steps include handling missing values, removing outliers, and normalizing data ranges.
Feature engineering plays a vital role in how to apply trading AI successfully, creating meaningful inputs for your models.
Building Your AI Trading Model
The process of building an AI trading model requires careful consideration of various factors and components.
Traders must understand how to apply trading AI architecture principles when designing their systems.
Selecting the right combination of algorithms and parameters can significantly impact model performance.
Regular testing and validation ensure your model maintains its predictive accuracy over time.
Model Training and Optimization
Training an AI model requires significant computational resources and a deep understanding of optimization techniques.
Learning how to apply trading AI training procedures effectively can mean the difference between success and failure.
Cross-validation helps ensure your model performs consistently across different market conditions.
Hyperparameter optimization fine-tunes your model’s performance to achieve maximum accuracy.
Implementation and Risk Management
Successfully implementing your AI trading model requires careful attention to risk management principles.
Understanding how to apply trading AI risk controls is essential for protecting your capital.
Position sizing, stop-loss orders, and portfolio diversification remain crucial components of any trading strategy.
Regular monitoring and adjustment of risk parameters ensure long-term sustainability.
Performance Monitoring and Adjustment
Continuous monitoring of your AI trading system’s performance is crucial for maintaining high accuracy.
Knowing how to apply trading AI monitoring tools helps identify potential issues before they become problems.
Regular model retraining and adjustment ensure your system stays aligned with current market conditions.
Documentation of system performance and modifications helps track improvements over time.
Advanced Techniques for Maximum Accuracy
Achieving 90% accuracy requires implementing advanced techniques and constant refinement.
Ensemble methods, combining multiple AI models, often provide more reliable predictions than single models.
Deep learning architectures can capture complex market patterns that simpler models might miss.
Understanding how to apply trading AI in combination with traditional technical analysis can enhance overall performance.
Real-Time Adaptation and Learning
Markets constantly evolve, requiring AI systems to adapt to changing conditions.
Implementing online learning capabilities allows your system to update in real-time.
Understanding how to apply trading AI adaptation techniques keeps your model current and effective.
Regular evaluation of model assumptions helps maintain prediction accuracy over time.
Conclusion
Mastering how to apply trading AI models requires dedication, technical knowledge, and continuous learning.
Success in AI-driven trading comes from understanding both the technology and the markets themselves.
Regular practice and refinement of your approach will help you achieve and maintain high prediction accuracy.
Remember that even the most sophisticated AI systems require human oversight and judgment.
Stay committed to learning and improving your understanding of how to apply trading AI techniques.
With proper implementation and management, achieving 90% accuracy in market prediction is possible.
Continue to explore new developments in AI trading technology and adapt your strategies accordingly.
Your journey in AI-driven trading is an ongoing process of learning, adaptation, and refinement.
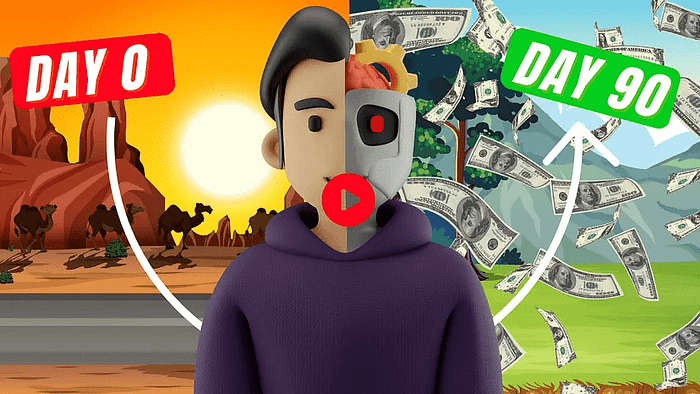
We strongly recommend that you check out our guide on how to take advantage of AI in today’s passive income economy.