Navigating the Future: Enterprise AI Management Solutions
Pioneering teams worldwide are transforming how they manage AI agent version control, creating a new standard for scalable artificial intelligence development.
The landscape of AI development has witnessed an unprecedented shift in how organizations track, manage, and scale their AI systems.
As businesses ranging from ambitious startups to established tech giants embrace AI technologies, the need for robust ai agent version control systems has become paramount.
These systems serve as the backbone of successful AI implementation, enabling teams to maintain consistency, track changes, and scale operations effectively.
The journey from a small startup to a tech giant requires careful consideration of how AI agents are versioned, deployed, and managed across different stages of growth.
Modern ai agent version control platforms offer sophisticated features that adapt to growing organizational needs while maintaining security and performance.
Understanding these systems is crucial for organizations aiming to build sustainable and scalable AI operations.
This comprehensive guide explores the most effective ai agent version control solutions that successfully scale with organizational growth.
We strongly recommend that you check out our guide on how to take advantage of AI in today’s passive income economy.
Table of Contents
Understanding AI Agent Version Control Fundamentals
Version control for AI agents differs significantly from traditional software version control systems, incorporating unique elements specific to AI development.
AI agent version control systems must handle complex dependencies, model weights, and training data versions simultaneously.
These specialized platforms ensure that every aspect of an AI agent’s development is tracked, from initial training to deployment.
The fundamental components include model versioning, dataset management, and prompt engineering history.
Organizations implementing ai agent version control benefit from improved collaboration, reduced errors, and enhanced reproducibility.
The ability to track changes across multiple AI agents and their respective versions becomes increasingly valuable as operations scale.
Modern systems incorporate features like branching, merging, and rollback capabilities specifically designed for AI development.
Understanding these fundamentals is essential for teams looking to implement scalable AI solutions effectively.
Key Features of Scalable AI Agent Version Control Systems
Successful ai agent version control platforms share several critical features that enable seamless scaling:
Distributed version control capabilities allow teams to work concurrently on multiple AI agents without conflicts.
Advanced metadata management tracks essential information about each version, including training parameters and performance metrics.
Automated testing and validation ensure consistency across different versions of AI agents.
Integration capabilities with popular development tools and frameworks streamline the workflow.
Security features protect sensitive AI models and training data while enabling controlled access across teams.
These platforms offer robust backup and recovery options to prevent data loss and maintain business continuity.
Real-time collaboration tools facilitate communication between team members working on different aspects of AI development.
The most effective systems provide comprehensive audit trails for compliance and governance requirements.
Popular AI Agent Version Control Solutions
Several leading platforms have emerged as frontrunners in the ai agent version control space:
ModelHub offers enterprise-grade version control specifically designed for large-scale AI operations.
VersionAI provides seamless integration with existing development workflows and tools.
AgentTrack focuses on specialized features for managing complex AI agent ecosystems.
These platforms have demonstrated their ability to scale effectively with growing organizations.
Each solution offers unique advantages and specializes in different aspects of AI agent management.
The choice of platform often depends on specific organizational needs and growth trajectories.
Regular updates and feature additions ensure these systems remain current with evolving AI technologies.
User feedback and community support contribute to continuous improvement of these platforms.
Implementation Strategies for Different Organization Sizes
Starting with ai agent version control requires careful planning and consideration of organizational needs:
Small startups should focus on essential features while maintaining flexibility for future growth.
Mid-sized organizations benefit from more robust features that support team collaboration.
Enterprise-level implementations require comprehensive solutions that handle complex deployment scenarios.
The implementation strategy should align with both current needs and future scaling plans.
Organizations must consider factors like team size, project complexity, and resource availability.
Training requirements and learning curves influence the success of implementation efforts.
Regular evaluation and adjustment of implementation strategies ensure optimal results.
Successful implementation often involves phased approaches that gradually introduce new features.
Scaling Considerations and Best Practices
As organizations grow, their ai agent version control needs evolve:
Infrastructure requirements change significantly when scaling from small teams to enterprise operations.
Security protocols must adapt to accommodate increased user access and data volume.
Performance optimization becomes crucial as the number of AI agents and versions increases.
Regular audits and updates ensure the system continues to meet organizational needs.
Documentation and standardization of processes support successful scaling efforts.
Team training and support resources must scale alongside technical capabilities.
Integration with other enterprise systems becomes increasingly important.
Organizations must balance flexibility and control as they scale their AI operations.
Future Trends in AI Agent Version Control
The future of ai agent version control systems shows promising developments:
Advanced automation features will streamline version management processes.
Improved integration with emerging AI technologies will enhance system capabilities.
Enhanced analytics and reporting tools will provide deeper insights into AI agent performance.
Machine learning techniques will optimize version control operations automatically.
Cloud-native solutions will offer improved scalability and accessibility.
New collaboration features will support globally distributed teams more effectively.
Integration with quantum computing platforms may revolutionize version control capabilities.
Organizations must stay informed about these trends to maintain competitive advantages.
Conclusion
The evolution of ai agent version control systems continues to shape how organizations manage and scale their AI operations.
Successful implementation requires careful consideration of organizational needs and growth plans.
Regular evaluation and updates ensure these systems continue to meet evolving requirements.
Organizations must choose solutions that provide both immediate benefits and long-term scalability.
The future of AI development depends heavily on robust version control systems.
Continued innovation in this space will drive improvements in AI agent management.
Organizations that effectively implement and scale these systems position themselves for success.
The journey from startup to tech giant becomes more manageable with the right version control solution in place.
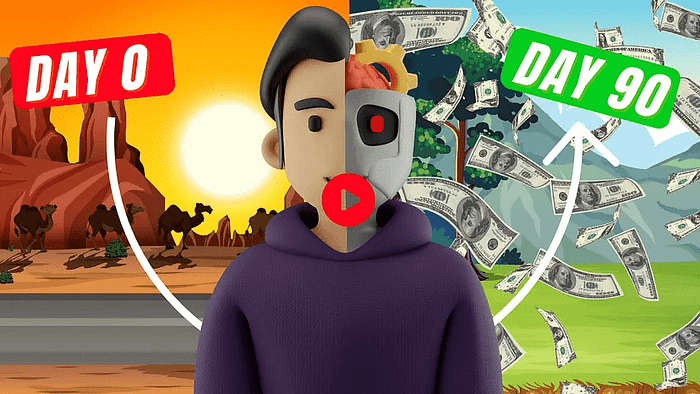
We strongly recommend that you check out our guide on how to take advantage of AI in today’s passive income economy.