What Andrew Ng Reveals About AI Agentic Workflows’ Future
AI agentic workflows are reshaping the landscape of artificial intelligence, propelling us into an era of unprecedented technological advancement.
As a researcher and AI enthusiast, I’ve been captivated by the rapid evolution of these intelligent systems.
The concept of AI agents working autonomously, making decisions, and iterating on tasks is no longer confined to science fiction.
It’s a reality that’s unfolding before our eyes, promising to transform industries and redefine human-machine interaction.
In this article, we’ll explore the cutting-edge developments in AI agentic workflows, delving into their potential impact and the exciting possibilities they bring to the table.
We strongly recommend that you check out our guide on how to take advantage of AI in today’s passive income economy.
Table of Contents
The Rise of AI Agentic Workflows
Understanding AI Agentic Workflows
AI agentic workflows represent a paradigm shift in how we interact with and utilize artificial intelligence systems.
Unlike traditional AI models that respond to prompts with static outputs, agentic workflows empower AI to think, plan, and execute tasks more dynamically.
This approach mimics human cognitive processes, allowing AI to break down complex problems, gather information, and iterate on solutions.
The result is a more flexible and powerful AI system capable of handling intricate tasks with greater efficiency and creativity.
The Limitations of Non-Agentic AI
To truly appreciate the power of AI agentic workflows, we must first understand the limitations of non-agentic systems.
Traditional AI models often operate in a linear fashion, generating responses based solely on the initial input they receive.
This approach, while effective for simple tasks, falls short when faced with complex, multi-step problems that require reasoning and adaptation.
Non-agentic AI lacks the ability to reflect on its own outputs, seek additional information, or refine its approach based on intermediate results.
The Transformative Power of AI Agentic Workflows
AI agentic workflows address these limitations by introducing a more sophisticated, iterative approach to problem-solving.
These systems can break down tasks into manageable steps, evaluate their progress, and adjust their strategies accordingly.
This level of autonomy and adaptability opens up new possibilities for AI applications across various industries.
From software development to scientific research, AI agentic workflows are poised to revolutionize how we approach complex challenges.
Key Components of AI Agentic Workflows
Reflection: The Foundation of Intelligent Decision-Making
At the heart of AI agentic workflows lies the crucial component of reflection.
This capability allows AI systems to analyze their own outputs, identify potential errors or areas for improvement, and refine their approach.
Reflection enables AI to engage in a form of self-critique, much like a human expert reviewing their own work.
This iterative process leads to higher quality results and more robust problem-solving capabilities.
Tool Use: Expanding AI Capabilities
Another vital aspect of AI agentic workflows is the integration of tool use.
By leveraging external tools and resources, AI agents can expand their capabilities beyond their core programming.
This might include accessing databases, performing web searches, or utilizing specialized algorithms for specific tasks.
Tool use allows AI to gather additional information and perform actions that would otherwise be beyond its reach.
Planning: Strategizing for Complex Tasks
Planning is a critical component that elevates AI agentic workflows to new heights of sophistication.
AI systems equipped with planning capabilities can break down complex tasks into logical sequences of actions.
This allows them to approach problems strategically, anticipating potential obstacles and devising solutions in advance.
The ability to plan enables AI to tackle more intricate challenges with greater efficiency and effectiveness.
Multi-Agent Collaboration: Harnessing Collective Intelligence
Perhaps one of the most exciting developments in AI agentic workflows is the emergence of multi-agent collaboration.
This approach involves multiple AI agents working together, each bringing specialized knowledge or skills to the table.
By simulating different roles or perspectives, these collaborative systems can generate more comprehensive and nuanced solutions.
Multi-agent collaboration opens up new possibilities for problem-solving and decision-making in complex domains.
The Impact of AI Agentic Workflows on Various Industries
Revolutionizing Software Development
AI agentic workflows are poised to transform the software development landscape.
By automating complex coding tasks and providing intelligent assistance to developers, these systems can significantly boost productivity.
Imagine an AI agent that can not only generate code but also test it, identify bugs, and propose optimizations—all in a fraction of the time it would take a human developer.
This level of automation and intelligence could accelerate innovation and reduce time-to-market for software products.
Advancing Scientific Research
In the realm of scientific research, AI agentic workflows offer unprecedented opportunities for data analysis and hypothesis generation.
These systems can sift through vast amounts of scientific literature, identify patterns, and propose novel research directions.
By automating time-consuming tasks like literature reviews and data processing, AI agents free up researchers to focus on higher-level thinking and experimentation.
The potential for accelerating scientific discoveries and breakthroughs is immense.
Enhancing Customer Service and Support
AI agentic workflows are set to revolutionize customer service and support industries.
Intelligent agents capable of understanding context, accessing relevant information, and collaborating with human agents can provide more personalized and efficient customer experiences.
These systems can handle complex queries, troubleshoot issues, and even anticipate customer needs proactively.
The result is improved customer satisfaction and reduced workload for human support staff.
Transforming Healthcare and Medical Diagnosis
In healthcare, AI agentic workflows have the potential to enhance medical diagnosis and treatment planning.
AI agents can analyze patient data, medical histories, and the latest research to assist healthcare professionals in making more informed decisions.
These systems can also collaborate with specialists across different medical fields, providing a more holistic approach to patient care.
The integration of AI agentic workflows in healthcare promises more accurate diagnoses, personalized treatment plans, and improved patient outcomes.
Challenges and Considerations
Ethical Implications of Autonomous AI Agents
As AI agentic workflows become more sophisticated and autonomous, we must grapple with important ethical considerations.
Questions arise about the accountability and transparency of decisions made by AI agents, especially in critical domains like healthcare or finance.
Ensuring that these systems operate within ethical boundaries and align with human values is paramount.
We must develop robust frameworks for governing AI agentic workflows to mitigate potential risks and build public trust.
The Need for Explainable AI in Agentic Systems
The complexity of AI agentic workflows presents challenges in terms of explainability and interpretability.
As these systems make decisions through intricate processes involving multiple agents and tools, it becomes crucial to understand their reasoning.
Developing methods for making AI agentic workflows more transparent and explainable is essential for their adoption in regulated industries.
This challenge calls for innovative approaches to AI design that balance performance with interpretability.
Balancing Autonomy and Human Oversight
Finding the right balance between AI autonomy and human oversight is a critical consideration in the development of AI agentic workflows.
While these systems offer immense potential for automation and efficiency, human judgment and expertise remain invaluable in many domains.
Striking the optimal balance requires careful thought about when and how human intervention should occur in AI-driven processes.
This challenge necessitates the development of collaborative frameworks that leverage the strengths of both AI and human intelligence.
The Future of AI Agentic Workflows
Emerging Trends and Possibilities
As we look to the future, several exciting trends are emerging in the field of AI agentic workflows.
Advancements in natural language processing are enabling more seamless human-AI collaboration, with agents capable of understanding and generating human-like text.
The integration of AI agentic workflows with other cutting-edge technologies like blockchain and the Internet of Things promises to create even more powerful and versatile systems.
We can expect to see AI agents taking on increasingly complex and creative tasks, from writing novels to designing innovative products.
The Path Towards More General AI
AI agentic workflows represent a significant step towards more general artificial intelligence.
By combining reflection, planning, tool use, and collaboration, these systems are beginning to exhibit more human-like problem-solving capabilities.
While true artificial general intelligence (AGI) remains a distant goal, the development of AI agentic workflows is paving the way for more flexible and adaptable AI systems.
This progress brings us closer to AI that can generalize knowledge across domains and tackle a wide range of real-world challenges.
Preparing for a Future Shaped by AI Agentic Workflows
As AI agentic workflows continue to evolve and shape our world, it’s crucial for individuals and organizations to prepare for this transformative technology.
Embracing AI literacy and developing skills that complement AI capabilities will be essential for thriving in this new landscape.
Organizations must also consider how to integrate AI agentic workflows into their existing processes and strategies to remain competitive.
By staying informed and adaptive, we can harness the full potential of AI agentic workflows to drive innovation and solve global challenges.
Conclusion
The rise of AI agentic workflows marks a new chapter in the evolution of artificial intelligence.
These sophisticated systems, capable of reflection, planning, and collaboration, are poised to revolutionize industries and redefine the boundaries of what AI can achieve.
As we navigate this exciting frontier, it’s crucial to address the ethical and practical challenges that come with more autonomous and powerful AI systems.
By doing so, we can ensure that AI agentic workflows serve as a force for positive change, augmenting human capabilities and driving progress across all sectors of society.
The future of AI is not just about smarter machines, but about creating intelligent systems that can work alongside humans to solve the world’s most pressing problems.
AI agentic workflows are a significant step towards realizing this vision, opening up new possibilities for innovation, discovery, and human-AI collaboration.
As we continue to explore and develop these technologies, we stand on the brink of a new era in artificial intelligence—one that promises to transform our world in ways we’re only beginning to imagine.
Frequently Asked Questions:
What is agentic AI?
Agentic AI refers to artificial intelligence systems that possess a degree of autonomy and decision-making capabilities.
These AI agents can operate independently to achieve specified goals, much like human agents would.
Agentic AI systems are designed to perceive their environment, make decisions, and take actions to accomplish tasks.
They can adapt to changing circumstances, learn from experience, and often collaborate with other AI agents or humans.
This type of AI goes beyond simple input-output models, exhibiting more complex, goal-oriented behavior.
Agentic AI is at the forefront of AI development, pushing the boundaries of what artificial intelligence can achieve.
What is the difference between agentic and non-agentic AI?
The primary distinction between agentic and non-agentic AI lies in their level of autonomy and decision-making capabilities.
Non-agentic AI systems typically operate on a simple input-output model, responding to specific prompts or queries.
These traditional AI models generate responses based solely on their initial programming and the input they receive.
In contrast, agentic AI systems have a higher degree of autonomy and can make decisions independently.
Agentic AI can break down complex tasks, plan strategies, and adapt their approach based on feedback and changing conditions.
While non-agentic AI excels at specific, predefined tasks, agentic AI is more flexible and capable of handling complex, multi-step problems.
What is an AI workflow?
An AI workflow refers to the sequence of steps and processes that an AI system follows to complete a task or achieve a goal.
It encompasses the entire journey from receiving an initial input or instruction to delivering the final output or result.
AI workflows can involve various stages such as data collection, processing, analysis, decision-making, and action execution.
In the context of agentic AI, workflows are often more complex and iterative, involving multiple feedback loops and decision points.
These workflows enable AI systems to tackle intricate problems by breaking them down into manageable steps and sub-tasks.
Understanding and optimizing AI workflows is crucial for developing more efficient and effective artificial intelligence systems.
What are the four stages of an AI workflow?
While AI workflows can vary depending on the specific application, four common stages are often identified:
- Data Ingestion: This initial stage involves collecting and preparing the necessary data for the AI system to work with. It may include gathering information from various sources, cleaning the data, and formatting it for analysis. In agentic AI workflows, this stage might also involve defining the problem and setting initial goals.
- Processing and Analysis: During this stage, the AI system processes the ingested data and performs various analyses. This can include applying machine learning algorithms, pattern recognition, or other analytical techniques. For agentic AI, this stage often involves planning and strategizing based on the available information.
- Decision Making: In this crucial stage, the AI system evaluates the results of its analysis and makes decisions. For agentic AI, this might involve choosing between different courses of action or refining its approach. The decision-making process can be based on predefined rules, learned patterns, or more complex reasoning algorithms.
- Action and Output: The final stage involves the AI system taking action based on its decisions or generating output. This could range from providing recommendations to executing specific tasks or commands. In agentic AI workflows, this stage might also include evaluating the results and initiating further iterations if necessary.
These four stages form a cycle that can be repeated or iterated upon, especially in more advanced AI agentic workflows.
Understanding these stages helps in designing more effective AI systems and optimizing their performance for various applications.
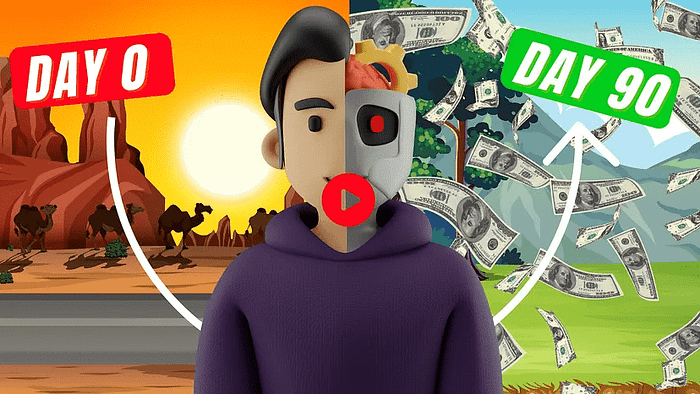
We strongly recommend that you check out our guide on how to take advantage of AI in today’s passive income economy.