The Future of AI Automation: What 99% of Businesses Don’t See Coming
AI automation future is transforming businesses at an unprecedented pace, revolutionizing how companies operate and scale their operations. A recent LinkedIn post caught my attention, highlighting how an AI agent built on Claude replaced a $100,000-per-year media buyer position, generating over 10,000 comments and sparking intense discussion about the future of work.
We strongly recommend that you check out our guide on how to take advantage of AI in today’s passive income economy.
Table of Contents
Understanding the AI Automation Revolution
The excitement surrounding AI automation future isn’t just hype – it’s backed by significant market indicators. Y Combinator, a leading startup accelerator, predicts that vertical AI agents could become ten times larger than the SaaS industry. This projection reflects a fundamental shift in how businesses approach automation and operational efficiency.
Defining AI Agents and Automation Systems
In the realm of AI automation future, understanding the distinction between various types of automation is crucial. An AI agent is a system that uses a large language model (LLM) to decide the control flow of an application. This definition might seem simple, but it encompasses a complex hierarchy of automation technologies.
The Automation Hierarchy
Basic Automation
At its foundation, we have simple actions executed through APIs. These are the building blocks of business process automation, connecting different tools and enabling basic task execution. Tools like Make.com and Zapier have made these connections accessible even to non-technical users.
AI-Enhanced Automation
The next level introduces AI processing into traditional automation workflows. While some might mistake this for true AI agents, it’s important to understand that these systems simply process information through AI models without making autonomous decisions about workflow direction.
Advanced AI Systems
Dynamic AI Automation
Dynamic AI automation represents a significant step forward, where AI systems make decisions about which actions to take based on available information. This creates what we call “agentic behavior” – the system shows agency in its decision-making process while maintaining predictable outcomes.
True AI Agents
A true AI agent combines decision-making capabilities with access to tools, memory, and vector databases. These systems can handle complex tasks by coordinating multiple tools and making sophisticated decisions based on context and previous interactions.
Multi-Agent Systems: The Future of Business Operations
The most advanced implementation of AI automation future involves multiple agents working together in coordinated systems. These can include:
- Prompt chaining workflows
- Parallelization workflows
- Routing workflows
- Orchestrator workflows
- Evaluator systems
Real-World Implementation Example
Consider an advertising campaign optimization system. Starting with basic automation for data collection, progressing through AI-enhanced analysis, and culminating in a multi-agent system that handles everything from performance monitoring to creative generation.
Implementation Framework for Businesses
Breaking Down Business Processes
Success with AI automation future requires methodical process documentation and analysis. Break down departmental responsibilities into specific tasks and actions, then evaluate each for automation potential.
The Optimization Hierarchy
Start with the simplest possible solution for each task:
- Basic automation where sufficient
- AI-enhanced automation where needed
- Dynamic AI systems for complex decisions
- Full AI agents for sophisticated coordination
- Multi-agent systems for complete process management
Current Challenges and Limitations
Recent industry reports indicate a 50/50 split between companies currently using AI agents in production and those in development phases. Key limitations include:
- Performance and quality concerns
- Cost considerations
- Safety and security issues
- System latency
Implementation Approaches
Technical Implementation
For organizations with technical capabilities, frameworks like LangChain provide robust foundations for building AI agents. The open-source community continues to develop tools and resources for agent development.
No-Code Solutions
Tools like n8n offer accessible entry points for businesses without extensive technical resources. These platforms provide built-in agentic frameworks while maintaining user-friendly interfaces.
Strategic Considerations for Business Leaders
When implementing AI automation future solutions, consider:
- Process documentation requirements
- System reliability needs
- Cost-benefit analysis
- Implementation timeline
- Training and oversight requirements
Conclusion
The AI automation future isn’t a distant possibility – it’s unfolding now. While complete business transformation through AI agents may not be immediate, the competitive advantage of early adoption is clear. Organizations that begin methodically implementing these technologies today will be better positioned for tomorrow’s business landscape.
Success in this transition requires careful planning, systematic implementation, and a clear understanding of both the potential and limitations of current AI agent technologies. The future belongs to those who can effectively combine human insight with AI capabilities, creating more efficient and effective business operations.
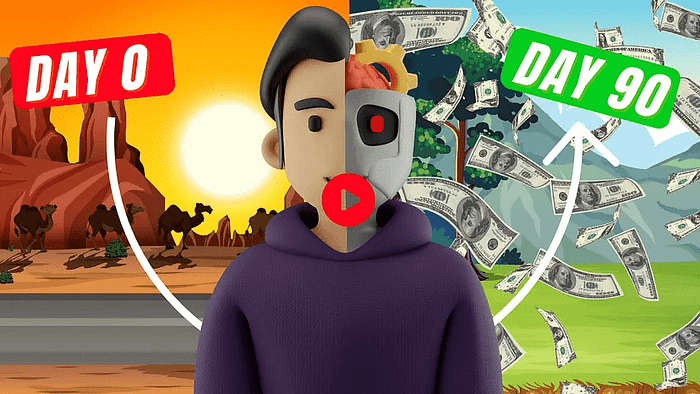
We strongly recommend that you check out our guide on how to take advantage of AI in today’s passive income economy.