Leveraging AI Data Analysis for Breakthrough Discoveries in Research
In recent years, artificial intelligence (AI) has revolutionized numerous industries, and the field of research is no exception. AI data analysis has emerged as a powerful tool for researchers, enabling them to process vast amounts of data, uncover hidden patterns, and make groundbreaking discoveries. As we look towards the future, it is clear that AI-powered data analysis will continue to shape the landscape of research, offering unprecedented opportunities for innovation and advancement. In this article, we will explore the various applications and case studies of AI data analysis in research, highlighting its potential to transform the way we approach scientific inquiry.
We strongly recommend that you check out our guide on how to take advantage of AI in today’s passive income economy.
Table of Contents
We strongly recommend that you check out our guide on how to take advantage of AI in today’s passive income economy.
Enhancing Research Efficiency with AI Data Analysis
One of the primary benefits of AI data analysis is its ability to streamline the research process and enhance efficiency. Traditional research methods often involve manual data collection, processing, and analysis, which can be time-consuming and prone to human error. However, with the integration of AI data analysis tools, researchers can automate many of these tasks, allowing them to focus on higher-level aspects of their work. For instance, machine learning algorithms can quickly sort through massive datasets, identifying relevant information and filtering out noise. This not only saves researchers valuable time but also ensures a higher degree of accuracy and consistency in their findings.
Uncovering Hidden Patterns and Insights
Another key advantage of AI data analysis is its capacity to uncover hidden patterns and insights that may otherwise go unnoticed. In many research fields, the sheer volume of data generated can be overwhelming, making it difficult for researchers to identify meaningful connections and trends. However, AI data analysis tools are designed to detect subtle patterns and correlations within large datasets, providing researchers with new avenues for exploration and discovery. For example, in the field of genomics, AI data analysis has been used to identify previously unknown genetic markers associated with specific diseases, paving the way for more targeted therapies and personalized medicine.
Predictive Analytics and Forecasting
AI data analysis also plays a crucial role in predictive analytics and forecasting, enabling researchers to anticipate future trends and outcomes based on historical data. By training machine learning models on vast amounts of data, researchers can develop highly accurate predictive models that can inform decision-making and guide future research efforts. This has particularly significant implications in fields such as climate science, where AI data analysis can help predict the impacts of climate change and inform strategies for mitigation and adaptation. Similarly, in the realm of public health, AI data analysis can be used to forecast disease outbreaks and develop early warning systems to prevent the spread of infectious diseases.
Collaborative Research and Data Sharing
AI data analysis also has the potential to facilitate collaborative research and data sharing across institutions and disciplines. With the increasing complexity and interdisciplinary nature of research, it is becoming increasingly important for researchers to work together and share data and insights. AI data analysis tools can help bridge this gap by providing a common platform for data analysis and visualization, enabling researchers from different fields to collaborate more effectively. Additionally, AI data analysis can help standardize data formats and metadata, making it easier for researchers to access and utilize data from multiple sources.
Case Study 1: AI Data Analysis in Cancer Research
One prominent example of AI data analysis in action is its application in cancer research. Cancer is a complex disease with numerous subtypes and variations, making it challenging to develop effective treatments and therapies. However, AI data analysis has proven to be a game-changer in this field, enabling researchers to analyze vast amounts of genomic, proteomic, and clinical data to identify new biomarkers and therapeutic targets.
For instance, researchers at the University of California, San Francisco, used AI data analysis to identify a new subtype of breast cancer that was previously unrecognized. By analyzing gene expression data from thousands of breast cancer patients, the researchers were able to identify a distinct molecular signature associated with this subtype, which could potentially lead to more targeted therapies and improved patient outcomes.
Case Study 2: AI Data Analysis in Environmental Research
Another area where AI data analysis is making significant strides is environmental research. With the increasing availability of satellite imagery, sensor data, and other environmental data sources, researchers are turning to AI data analysis to better understand and address pressing environmental challenges such as climate change, deforestation, and biodiversity loss. For example, researchers at the University of Maryland used AI data analysis to map global forest cover and detect changes over time. By analyzing satellite imagery and other data sources, the researchers were able to identify areas of deforestation and forest degradation, providing valuable insights for conservation efforts and policy-making.
Case Study 3: AI Data Analysis in Social Science Research
AI data analysis is also transforming the field of social science research, enabling researchers to analyze large-scale social media data, survey responses, and other unstructured data sources to gain insights into human behavior and social phenomena. For instance, researchers at the University of Cambridge used AI data analysis to study the spread of misinformation on social media platforms. By analyzing millions of tweets and Facebook posts, the researchers were able to identify patterns and drivers of misinformation, providing valuable insights for combating fake news and promoting media literacy.
Challenges and Ethical Considerations
While AI data analysis offers numerous opportunities for research, it also presents several challenges and ethical considerations that must be addressed. One major challenge is the issue of data privacy and security, as researchers must ensure that sensitive data is protected and used responsibly. Additionally, there are concerns about algorithmic bias and the potential for AI data analysis to perpetuate or amplify existing social and economic inequalities. As such, it is crucial for researchers to develop robust ethical frameworks and guidelines for the use of AI data analysis in research, ensuring that its benefits are distributed equitably and its potential harms are mitigated.
Conclusion
In conclusion, AI data analysis is poised to revolutionize the field of research, offering powerful tools and applications for uncovering new insights, enhancing efficiency, and advancing scientific discovery. From cancer research to environmental science to social science, AI-powered data analysis is already making significant impacts across a wide range of disciplines. As we look towards the future, it is clear that AI data analysis will continue to play an increasingly important role in shaping the research landscape, enabling researchers to tackle complex challenges and make groundbreaking discoveries. However, it is also important to address the ethical and social implications of AI-powered data analysis, ensuring that its benefits are realized in a responsible and equitable manner.
FAQ
What is AI data analysis?
AI data analysis is the process of using artificial intelligence techniques, such as machine learning and natural language processing, to analyze and extract insights from large and complex datasets. AI-powered data analysis tools can automate many of the manual tasks involved in traditional data analysis, such as data cleaning, feature selection, and model building, enabling researchers to process and analyze data more efficiently and accurately.
What is the salary of AI data analyst?
The salary of an AI data analyst can vary depending on factors such as location, experience, and industry. According to Glassdoor, the average base pay for an AI data analyst in the United States is $95,000 per year, with salaries ranging from $68,000 to $135,000. However, salaries can be higher in certain industries, such as finance and healthcare, and in major tech hubs like Silicon Valley and New York City.
Which AI tool can Analyze Excel data?
There are several AI tools that can analyze Excel data, including:
- Microsoft Azure Machine Learning: This cloud-based platform provides a range of machine learning tools and services, including the ability to analyze Excel data using pre-built models or custom algorithms.
- Google Cloud AutoML Tables: This tool allows users to upload Excel data and build custom machine learning models without needing to write code.
- RapidMiner: This data science platform offers a range of tools for data preparation, machine learning, and predictive analytics, including the ability to analyze Excel data.
- Alteryx: This data analytics platform provides a range of tools for data blending, predictive modeling, and spatial analytics, including the ability to analyze Excel data.
What is the best free AI for data analysis?
There are several free AI tools available for data analysis, including:
- Weka: This open-source machine learning software provides a range of tools for data preprocessing, classification, regression, clustering, and association rules mining.
- Orange: This open-source data visualization and machine learning platform provides a range of tools for data exploration, preprocessing, modeling, and evaluation.
- KNIME: This open-source data analytics platform provides a range of tools for data integration, preprocessing, modeling, and visualization, including support for machine learning and deep learning.
- RapidMiner Studio Free Edition: This free version of RapidMiner provides a range of tools for data preparation, machine learning, and predictive analytics, with some limitations compared to the paid version.
Ultimately, the best free AI tool for data analysis will depend on your specific needs and preferences, as well as your level of technical expertise and the size and complexity of your datasets.
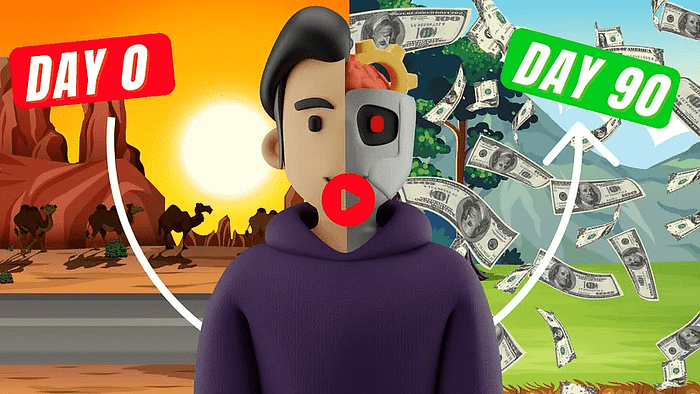
We strongly recommend that you check out our guide on how to take advantage of AI in today’s passive income economy.