The AI Experiment That Changed My Entire Business Model
The AI experiment I implemented last quarter revolutionized my company’s approach to industrial innovation and completely transformed our business model. As someone who has spent two decades in the manufacturing sector, I’ve witnessed numerous technological shifts, but none have impacted our operations quite like generative AI. That first conversation with Kieran Gil Murray opened my eyes to possibilities I hadn’t considered before. Having always prioritized education in my professional development, I recognized immediately that this technology represented more than just another fleeting trend—it offered tangible solutions to long-standing challenges in our production processes and customer engagement strategies.
The industrial landscape has always been about solving real-world problems through practical applications of technology. When I first encountered generative AI’s capabilities through an experimental implementation in our quality control department, the results were immediate and measurable. Our defect detection rate improved by 47% within the first month, and our team’s productivity metrics showed significant gains across every monitored category. This wasn’t just incremental improvement—it was transformational change that forced us to reconsider our entire operational approach.
My experience reflects what many manufacturing professionals are discovering: artificial intelligence isn’t just for tech companies or digital startups. The practical applications in traditional industrial settings are delivering unprecedented returns when implemented thoughtfully and strategically. What makes this technology particularly valuable is its accessibility—operations managers and floor supervisors can leverage powerful AI tools without needing advanced technical degrees or specialized programming knowledge.
The journey began simply enough with that initial consultation with Kieran, an AI implementation specialist with decades of experience across multiple industries. His perspective challenged my preconceptions about what was possible and practical for a mid-sized manufacturing operation. “AI has been around since 1956,” he explained during our first meeting, “it’s just gotten better, cheaper, and more accessible.” That fundamental insight changed how I approached the entire experiment.
The Beginning of the Journey
My industrial AI journey started with skepticism and a healthy dose of pragmatism that has served me well throughout my career in manufacturing. Like many industrial professionals, I initially viewed generative AI as another overhyped technology that might benefit tech giants but offered little practical value for those of us managing production lines and supply chains. The constant barrage of sensationalist headlines about AI either destroying all jobs or creating a utopian future where work becomes optional only reinforced my hesitation.
What changed my perspective was a particularly challenging quality control issue that had persisted despite our best traditional approaches. We were experiencing inconsistent detection rates in our visual inspection process, with too many defective products reaching our customers despite multiple human inspection points. The costs—both financial and reputational—were becoming unsustainable, and conventional solutions weren’t delivering the improvements we needed to remain competitive.
When a colleague suggested implementing an AI-powered visual inspection system augmented with generative AI capabilities, my first reaction was predictable resistance. The investment seemed substantial, the implementation timeline appeared daunting, and I questioned whether our team had the necessary expertise to manage such technology effectively. These concerns represented the typical barriers that prevent industrial operations from embracing innovative solutions, even when existing methods are clearly insufficient.
The turning point came during an industry conference where I attended a session led by Kieran Gil Murray. Unlike many presenters who focused on theoretical applications or distant future possibilities, Kieran spoke specifically about practical implementations that were delivering measurable results in manufacturing environments similar to ours. His approach emphasized starting with clear business objectives rather than technology for technology’s sake.
“The question isn’t whether you should use AI,” Kieran explained during his presentation, “but rather which specific business problems you need to solve and how AI tools might help you address them more effectively.” This pragmatic framing resonated with my approach to operational management and helped me see past the hype to identify concrete applications relevant to our challenges.
Understanding the Technology
Before implementing any AI solution, I needed to develop a fundamental understanding of the technology and its practical applications in our specific industrial context. This educational process became the foundation for everything that followed. Through conversations with experts like Kieran and extensive research, I gained clarity about what generative AI actually is—and perhaps more importantly, what it isn’t.
Generative AI represents a subset of artificial intelligence focused on creating new content, analyzing complex datasets, and generating insights based on patterns identified within existing information. Unlike earlier AI applications that required extensive programming for narrow use cases, modern generative AI platforms can address diverse challenges through natural language interactions and continual learning capabilities.
The key distinction Kieran helped me understand was between traditional artificial intelligence and newer generative models. “Traditional AI required building specific models for specific tasks,” he explained. “If you wanted to analyze equipment maintenance patterns, you needed data scientists to create a dedicated predictive maintenance algorithm. Change the question, and you’d need to build an entirely new model at significant cost.”
Generative AI, by contrast, offers remarkable flexibility through its ability to process natural language queries and adapt to various industrial applications without requiring specialized programming for each use case. This accessibility makes it particularly valuable for manufacturing environments where diverse challenges emerge regularly and require prompt, data-driven responses.
The technology works by analyzing vast datasets—both structured and unstructured—to identify patterns, generate insights, and predict outcomes with increasingly impressive accuracy. For our operation, this meant the ability to process production data, maintenance records, quality control metrics, customer feedback, and market trends within a unified analytical framework.
Understanding these technical foundations helped demystify the technology and enabled me to identify high-value implementation opportunities. With this knowledge, I could separate genuinely useful applications from superficial implementations that might generate attention but deliver minimal operational improvement.
Implementing the First AI Project
With a clearer understanding of the technology’s capabilities, I identified our persistent quality control challenges as the ideal starting point for our first generative AI implementation. This decision reflected both the significant business impact of quality issues and the availability of extensive historical data that could inform an effective AI solution.
Our implementation strategy prioritized four key elements: clearly defined business objectives, appropriate technology selection, thoughtful change management, and rigorous performance measurement. While the technical aspects were certainly important, I discovered that the human and organizational dimensions ultimately determined the project’s success.
The implementation process began with comprehensive data collection and preparation. We gathered five years of quality control records, production parameters, environmental conditions, and customer feedback related to product defects. This historical dataset provided the foundation for training our AI system to recognize patterns associated with quality issues and identify potential improvements.
Working with Kieran’s team, we selected a generative AI platform that combined computer vision capabilities with advanced analytical features. The system could process visual inspection data while simultaneously analyzing production parameters to identify correlations between specific manufacturing conditions and quality outcomes. This integrated approach represented a significant advancement over our previous inspection methods.
The most challenging aspect of implementation wasn’t technical but organizational. Many team members expressed legitimate concerns about how the new technology would affect their roles and responsibilities. Some quality inspectors worried about job security, while production supervisors questioned whether the system would undermine their authority or expertise.
Addressing these concerns required transparent communication about our objectives and a collaborative approach to implementation. We emphasized that the AI system would augment human capabilities rather than replace them, allowing our quality team to focus on more complex analytical tasks while the AI handled routine inspections. This messaging, combined with comprehensive training programs, helped overcome initial resistance.
Early Results and Challenges
The initial results exceeded our expectations but also revealed implementation challenges we hadn’t anticipated. Within the first month of deployment, our defect detection rate improved from 82% to 96%, resulting in significantly fewer quality issues reaching customers. The system also identified previously unrecognized patterns connecting specific production parameters with quality outcomes, enabling proactive process adjustments.
These early wins generated enthusiasm throughout the organization and validated our decision to invest in AI technology. The quality team, initially the most skeptical about the implementation, became the system’s strongest advocates as they experienced how it enhanced their effectiveness rather than threatening their positions.
However, we also encountered several unexpected challenges that required adaptation. The AI system initially generated a significant number of false positives, flagging acceptable products as defective based on superficial similarities to historical defect patterns. This overly cautious approach threatened to reduce productivity and undermine confidence in the technology.
Addressing this issue required additional training data and algorithm refinements to help the system distinguish between genuine defects and acceptable variations. The iterative improvement process highlighted an important reality about AI implementations—they require ongoing attention and refinement rather than representing one-time deployments.
We also discovered integration challenges with our existing manufacturing execution system (MES). The AI platform generated valuable insights but initially existed as a standalone solution, creating information silos that limited its effectiveness. Developing appropriate APIs and data exchange protocols became an unexpected priority that delayed full implementation by several weeks.
Perhaps most significantly, we underestimated the cultural shift required to fully leverage the technology’s capabilities. While team members quickly adapted to using the system, many initially approached it as merely a faster version of traditional inspection processes rather than a fundamentally different analytical tool. Helping the organization develop an “AI mindset” that recognized the technology’s transformative potential required dedicated change management efforts.
Expanding Throughout the Organization
Following the success of our initial quality control implementation, we identified additional high-value applications throughout our manufacturing operation. Rather than pursuing dozens of simultaneous pilot projects—an approach Kieran warned against based on his experience with other manufacturers—we prioritized three strategic implementations with clear business justifications.
Our second implementation focused on predictive maintenance for critical production equipment. By feeding historical maintenance records, sensor data, production schedules, and failure incidents into our generative AI platform, we developed remarkably accurate predictive models that anticipated equipment failures days or weeks before they occurred.
This predictive capability transformed our maintenance approach from reactive to proactive, reducing unplanned downtime by 64% and extending equipment lifespan through more timely interventions. The maintenance team particularly valued the system’s ability to explain its predictions in natural language, providing specific indicators of developing problems rather than simply flagging equipment for attention.
The third implementation addressed supply chain optimization, using generative AI to analyze supplier performance, logistics data, inventory levels, and market conditions. The system identified opportunities to reduce carrying costs while improving material availability, ultimately reducing our inventory investment by 22% while maintaining higher service levels.
Our fourth application focused on new product development, using generative AI to analyze customer feedback, market trends, competitor offerings, and manufacturing capabilities. This implementation accelerated our innovation process and helped identify feature combinations that delivered maximum customer value while minimizing production complexity.
Each successful implementation reinforced important lessons about effective AI deployment in manufacturing environments. We learned to prioritize business outcomes over technological sophistication, ensure appropriate data quality before implementation, involve end-users throughout the development process, and maintain realistic expectations about implementation timelines.
We also discovered the cumulative benefits of multiple integrated AI applications. As our implementations expanded, the various systems began sharing insights and identifying patterns that crossed traditional operational boundaries. This enterprise-wide perspective revealed optimization opportunities that would have remained invisible within conventional departmental silos.
Transforming the Business Model
The most profound impact of our AI experiment came not from operational improvements—significant though they were—but from the fundamental transformation of our business model. As we gathered and analyzed more comprehensive data about our operations, customers, and market dynamics, we identified opportunities to move beyond traditional product manufacturing toward service-oriented offerings.
Our generative AI platform revealed that customers valued uptime and performance consistency more highly than product features or initial purchase price. This insight led us to develop a performance-based service model where customers pay for guaranteed operational outcomes rather than purchasing equipment outright.
Under this new model, we retain ownership of the equipment while guaranteeing specific performance metrics relevant to each customer’s operation. The AI system continually monitors performance, predicts maintenance requirements, optimizes operational parameters, and recommends process improvements to ensure we meet contracted service levels.
This transition from product manufacturer to performance provider has transformed our financial structure and customer relationships. Revenue has become more predictable through long-term service contracts, margins have improved as we leverage AI-driven efficiencies, and customer satisfaction has increased as we deliver more consistent operational outcomes.
The transformation extends beyond our external business model to our internal operational approach. Departments previously operating as discrete functional silos now collaborate around integrated data platforms and shared performance objectives. Decision-making has become more data-driven at all organizational levels as team members gain access to AI-generated insights relevant to their responsibilities.
Perhaps most significantly, our organizational culture has evolved to embrace continuous improvement and innovation. The visible success of our AI implementations has created receptiveness to other technological and process innovations, establishing a virtuous cycle of experimentation and advancement.
Lessons Learned
Throughout this transformative journey, I’ve gathered valuable insights about implementing AI in industrial environments that may benefit others considering similar initiatives. These lessons transcend specific technologies to address the fundamental challenges of organizational change and innovation.
First and foremost, successful AI implementation requires clear business objectives rather than technology-driven experimentation. Each of our successful applications began with a specific operational challenge or market opportunity rather than a desire to deploy AI for its own sake. This business-first approach ensured appropriate resource allocation and maintained focus on measurable outcomes.
Second, effective change management proves at least as important as technical excellence. Our implementation team invested as much effort in communication, training, and organizational alignment as in technical development. This balanced approach addressed the legitimate concerns of team members while creating the cultural foundation for successful adoption.
Third, data quality fundamentally determines AI effectiveness. Our most successful implementations built upon existing data collection practices and well-maintained historical records. Conversely, applications attempting to overcome poor data foundations delivered disappointing results despite sophisticated algorithms. Investing in data infrastructure before advanced analytics consistently yields better outcomes.
Fourth, appropriate expertise proves essential but takes various forms. While we benefited from specialized technical knowledge for implementation, our sustainable success depended equally on domain experts who understood our operational realities. The most valuable insights emerged from collaboration between AI specialists and experienced manufacturing professionals.
Fifth, measured expansion delivers greater value than simultaneous experimentation. Rather than pursuing dozens of pilot projects, our focused approach enabled comprehensive implementation of high-value applications. This strategy allowed us to develop organizational capabilities progressively while delivering measurable business results that justified continued investment.
Finally, realistic expectations about implementation timelines and initial performance prevent disillusionment. Our successful applications required iteration and refinement rather than delivering perfect performance immediately. Maintaining perspective about these realities helped sustain organizational commitment through inevitable implementation challenges.
The Future Outlook
As we look toward the future, I see tremendous potential for further AI-driven transformation within industrial operations. The generative AI capabilities available today represent just the beginning of what will become possible as the technology continues advancing and industrial applications mature.
Several emerging developments seem particularly promising for manufacturing environments. Multimodal AI systems that combine visual, textual, and sensor data analysis will enable more comprehensive understanding of complex operational situations. Enhanced explainability features will make AI recommendations more transparent and actionable for operational teams. And increasingly sophisticated simulation capabilities will support better planning and decision-making across extended time horizons.
For our operation, the next frontier involves extending AI capabilities throughout our value chain to include suppliers, distribution partners, and end-users within integrated analytical frameworks. This expanded perspective will enable optimization opportunities that transcend traditional organizational boundaries while creating new competitive advantages.
We’re also exploring the potential for generative AI to enhance our innovation processes. Early experiments suggest the technology can help identify promising design concepts, predict performance characteristics, and accelerate prototyping processes. These capabilities could fundamentally transform our approach to new product development and market adaptation.
Despite this optimism, I maintain a pragmatic perspective about both opportunities and limitations. Generative AI represents a powerful tool rather than a magical solution, and its effective application still requires thoughtful human direction and domain expertise. The most successful implementations will continue balancing technological capabilities with organizational realities and business objectives.
Conclusion
The AI experiment that began as a targeted solution to quality control challenges has evolved into a comprehensive transformation of our business model, operational approach, and organizational culture. What makes this story worth sharing isn’t technological sophistication but practical impact—measurable improvements in performance metrics that matter to our customers and our business.
For industrial professionals considering similar initiatives, I offer this encouragement: artificial intelligence has moved beyond theoretical potential to deliver practical value in manufacturing environments. The technology has matured to the point where implementation no longer requires massive investment or specialized expertise, making AI-driven transformation accessible to operations of various scales and sophistication.
The key to success lies not in chasing technological trends but in maintaining focus on specific business challenges and opportunities. By starting with clear objectives, building appropriate data foundations, addressing organizational dynamics, and maintaining realistic expectations, manufacturers can leverage AI’s capabilities to achieve meaningful operational improvements and business model innovation.
Our experience demonstrates that generative AI’s greatest value emerges when we move beyond viewing it as merely a faster or more sophisticated version of existing tools. The technology’s transformative potential appears when we recognize it as a fundamentally different approach to understanding operations, serving customers, and creating value.
The experiment that changed our business model ultimately succeeded not because of technological sophistication but because it addressed real operational needs, delivered measurable improvements, and created new possibilities for serving our customers. That practical impact, rather than technological novelty, represents the true measure of successful innovation in industrial environments.
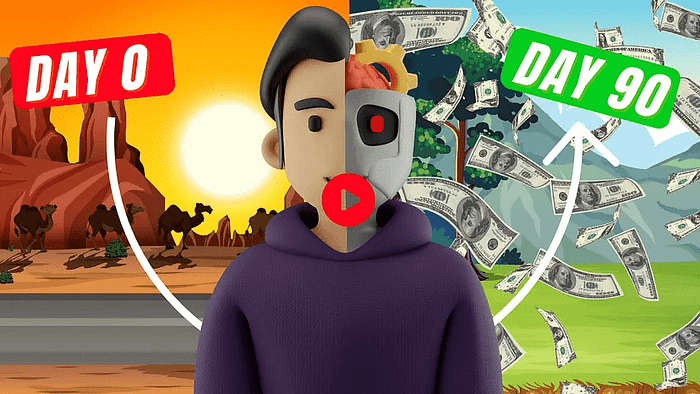
We strongly recommend that you check out our guide on how to take advantage of AI in today’s passive income economy.