How AI Tools in Finance Are Reshaping Investment Strategies
The rapid advancement of ai tools in finance has ushered in a new era of investment strategies, revolutionizing the way financial institutions and individual investors approach market analysis, risk management, and decision-making processes.
As technology continues to evolve at an unprecedented pace, the integration of artificial intelligence into the financial sector has become not just a competitive advantage but a necessity for those seeking to stay ahead in the ever-changing landscape of global markets.
This article will explore the profound impact of AI tools in finance on investment strategies, examining how these cutting-edge technologies are transforming traditional approaches and opening up new possibilities for investors of all levels.
From machine learning algorithms that can predict market trends with remarkable accuracy to natural language processing systems that can analyze vast amounts of financial news and data in real-time, the potential applications of AI in finance are both diverse and far-reaching.
We will delve into the various ways in which ai tools in finance are being utilized to enhance investment strategies, improve risk assessment, and ultimately drive better financial outcomes for individuals and institutions alike.
By understanding the capabilities and limitations of these advanced technologies, investors can make more informed decisions about how to incorporate AI into their own investment approaches and stay competitive in an increasingly technology-driven financial world.
Throughout this exploration, we will consider both the benefits and potential challenges associated with the growing reliance on AI tools in finance, as well as the implications for the future of investment strategies and the broader financial industry.
We strongly recommend that you check out our guide on how to take advantage of AI in today’s passive income economy.
Table of Contents
The Rise of AI in Financial Markets
Historical Context and Technological Evolution
The integration of ai tools in finance is not an entirely new phenomenon, but rather the culmination of decades of technological advancement and increasing computational power.
Since the early days of computerized trading systems in the 1970s, financial institutions have been at the forefront of adopting new technologies to gain a competitive edge in the markets.
However, it is only in recent years that the true potential of AI in reshaping investment strategies has begun to be realized, thanks to breakthroughs in machine learning, big data analytics, and cloud computing.
The exponential growth in data generation and storage capabilities has provided the fuel necessary for AI systems to learn, adapt, and make increasingly sophisticated predictions and decisions.
As a result, ai tools in finance have evolved from simple rule-based systems to complex neural networks capable of identifying patterns and insights that would be impossible for human analysts to discern on their own.
This technological evolution has fundamentally altered the landscape of financial markets, creating new opportunities for those who can effectively harness the power of AI while also posing challenges for traditional investment approaches that may struggle to keep pace with these rapid advancements.
Key AI Technologies Driving Change in Finance
Several key AI technologies are at the forefront of reshaping investment strategies in the financial sector.
Machine learning algorithms, particularly deep learning neural networks, have proven exceptionally adept at analyzing vast amounts of financial data and identifying complex patterns that can inform investment decisions.
Natural language processing (NLP) systems have revolutionized the way financial institutions process and interpret news, social media sentiment, and other textual data sources, providing valuable insights into market trends and potential investment opportunities.
Computer vision technology is being applied to analyze visual data such as satellite imagery and foot traffic patterns, offering unique perspectives on economic activity and consumer behavior that can inform investment strategies.
Reinforcement learning algorithms are being used to develop sophisticated trading strategies that can adapt to changing market conditions in real-time, optimizing portfolio performance and risk management.
These ai tools in finance, along with other emerging technologies such as quantum computing and blockchain, are collectively reshaping the landscape of investment strategies and challenging traditional notions of how financial markets operate.
As these technologies continue to evolve and become more widely adopted, their impact on investment strategies is likely to become even more pronounced, potentially leading to a paradigm shift in how financial decisions are made and executed.
Transforming Investment Analysis and Decision-Making
Enhanced Market Prediction and Trend Analysis
One of the most significant ways in which ai tools in finance are reshaping investment strategies is through their ability to enhance market prediction and trend analysis.
Traditional methods of market analysis often rely on historical data and human intuition, which can be limited in their ability to account for the complex, interconnected nature of global financial markets.
AI-powered predictive models, on the other hand, can process vast amounts of data from diverse sources, including market prices, economic indicators, geopolitical events, and even social media sentiment, to generate more accurate and timely forecasts.
These advanced predictive capabilities allow investors to anticipate market movements with greater precision, identify emerging trends before they become widely recognized, and make more informed decisions about when to enter or exit positions.
Moreover, AI systems can continuously learn and adapt their models based on new data and market conditions, ensuring that their predictions remain relevant and accurate even in rapidly changing environments.
This dynamic approach to market analysis enables investors to stay ahead of the curve and capitalize on opportunities that may be overlooked by traditional analytical methods.
As ai tools in finance continue to improve in their predictive capabilities, they are likely to become an increasingly essential component of successful investment strategies across all asset classes and market segments.
Automated Portfolio Management and Optimization
Another key area where ai tools in finance are making a significant impact is in automated portfolio management and optimization.
Traditional portfolio management often involves manual rebalancing and periodic adjustments based on predetermined rules or human judgment, which can be time-consuming and subject to bias or error.
AI-powered portfolio management systems, however, can continuously monitor market conditions, individual asset performance, and risk factors to make real-time adjustments that optimize portfolio allocation and performance.
These systems can analyze vast amounts of historical and real-time data to identify optimal asset allocations based on an investor’s specific goals, risk tolerance, and market conditions.
By leveraging machine learning algorithms, AI tools can also adapt their strategies over time, learning from past performance and market behavior to improve future decision-making.
This automated approach to portfolio management not only increases efficiency and reduces the potential for human error but also allows for more dynamic and responsive investment strategies that can quickly adapt to changing market conditions.
Furthermore, AI-driven portfolio optimization can help investors achieve better risk-adjusted returns by identifying and exploiting market inefficiencies and correlations that may not be apparent through traditional analysis.
As these ai tools in finance become more sophisticated and widely adopted, they have the potential to democratize access to advanced portfolio management techniques, making professional-grade investment strategies available to a broader range of investors.
Risk Assessment and Management
AI tools in finance are also revolutionizing the way investors approach risk assessment and management, providing more sophisticated and nuanced analyses of potential risks and opportunities.
Traditional risk management often relies on historical data and simplified models that may not adequately capture the complexity of modern financial markets or account for rare but impactful events.
AI-powered risk assessment tools, however, can analyze vast amounts of data from diverse sources to identify potential risks and vulnerabilities that may not be apparent through conventional analysis.
These systems can simulate countless scenarios and stress test portfolios under a wide range of market conditions, providing investors with a more comprehensive understanding of their risk exposure.
Machine learning algorithms can also detect subtle patterns and correlations in market data that may signal emerging risks or opportunities, allowing investors to take proactive measures to protect their portfolios or capitalize on market inefficiencies.
Furthermore, AI tools can continuously monitor and analyze real-time market data, news, and other relevant information to provide early warning signals of potential risks or market disruptions.
This enhanced risk management capability enables investors to make more informed decisions about asset allocation, hedging strategies, and overall portfolio construction, potentially leading to improved risk-adjusted returns and greater financial stability.
As ai tools in finance continue to evolve in their risk assessment capabilities, they are likely to become an indispensable component of robust investment strategies across all levels of the financial industry.
Sentiment Analysis and Alternative Data Processing
One of the most innovative ways in which ai tools in finance are reshaping investment strategies is through advanced sentiment analysis and the processing of alternative data sources.
Traditional investment analysis typically relies on structured financial data such as earnings reports, economic indicators, and market prices.
However, AI-powered sentiment analysis tools can now process vast amounts of unstructured data from sources such as news articles, social media posts, and online forums to gauge market sentiment and identify potential investment opportunities or risks.
Natural language processing algorithms can analyze the tone, context, and relevance of textual data, providing investors with valuable insights into market trends, company reputations, and potential catalysts for price movements.
Moreover, AI tools are enabling the integration of alternative data sources that were previously difficult or impossible to incorporate into investment strategies.
These may include satellite imagery to assess retail foot traffic or crop yields, credit card transaction data to gauge consumer spending trends, or web scraping to monitor pricing and inventory levels across e-commerce platforms.
By leveraging these diverse and often real-time data sources, AI-powered investment strategies can gain a more comprehensive and timely understanding of market dynamics and company performance.
This ability to process and derive insights from alternative data sources gives investors using ai tools in finance a significant edge in identifying investment opportunities and managing risks in an increasingly complex and fast-paced financial landscape.
Challenges and Considerations
Ethical and Regulatory Implications
As ai tools in finance continue to reshape investment strategies, they also raise important ethical and regulatory considerations that must be carefully addressed.
One of the primary concerns is the potential for AI systems to perpetuate or exacerbate existing biases in financial markets, particularly if the data used to train these systems reflects historical inequalities or discriminatory practices.
There are also questions about the transparency and explainability of AI-driven investment decisions, as the complex algorithms underlying these systems can often be difficult for humans to interpret or audit.
Regulatory bodies around the world are grappling with how to effectively oversee and govern the use of AI in financial markets, balancing the need for innovation with the imperative to protect investors and maintain market integrity.
Issues of data privacy and security also come to the forefront, as AI systems often require access to vast amounts of potentially sensitive financial and personal information.
Furthermore, the increasing reliance on AI tools in finance raises questions about the changing nature of work in the financial sector and the potential for job displacement as certain tasks become automated.
As these technologies continue to evolve and become more prevalent, it will be crucial for policymakers, industry leaders, and ethicists to work together to develop robust frameworks and guidelines for the responsible development and deployment of AI in investment strategies.
Addressing these ethical and regulatory challenges will be essential to ensuring that the benefits of AI in finance are realized while minimizing potential risks and negative consequences.
Limitations and Potential Pitfalls
While ai tools in finance offer tremendous potential for reshaping investment strategies, it is important to recognize their limitations and potential pitfalls.
One significant challenge is the risk of over-reliance on AI systems, which may lead to a false sense of security or overconfidence in their predictions and recommendations.
AI models, no matter how sophisticated, are ultimately based on historical data and patterns, and may struggle to accurately predict or respond to unprecedented events or market conditions.
There is also the risk of “black box” decision-making, where the rationale behind AI-generated investment strategies becomes opaque or difficult to explain, potentially leading to a lack of accountability and increased regulatory scrutiny.
Another limitation is the potential for AI systems to be vulnerable to adversarial attacks or manipulation, where bad actors intentionally feed misleading data or exploit weaknesses in the algorithms to influence market behavior.
The high cost and technical expertise required to develop and maintain advanced AI systems may also create barriers to entry, potentially exacerbating wealth inequality by giving larger institutions an unfair advantage over smaller investors.
Furthermore, the rapid pace of technological advancement in AI may lead to a “arms race” in financial markets, where the focus shifts from fundamental analysis and long-term value creation to short-term technological advantages.
As investors and financial institutions increasingly adopt ai tools in finance, it will be crucial to remain aware of these limitations and potential pitfalls, and to develop strategies to mitigate their impact on investment outcomes and market stability.
The Future of AI in Investment Strategies
Looking ahead, the role of ai tools in finance in reshaping investment strategies is likely to become even more pronounced and transformative.
As AI technologies continue to advance, we can expect to see even more sophisticated and powerful tools emerge, capable of processing increasingly complex data sets and making more nuanced investment decisions.
The integration of AI with other emerging technologies such as blockchain, quantum computing, and the Internet of Things is likely to open up new frontiers in financial analysis and investment strategy development.
We may see the rise of fully autonomous AI investment systems that can operate with minimal human intervention, potentially democratizing access to sophisticated investment strategies for a broader range of investors.
However, this future also brings challenges, including the need for ongoing education and skill development to keep pace with technological advancements, and the importance of maintaining human oversight and ethical considerations in AI-driven investment decisions.
The regulatory landscape will likely continue to evolve to address the unique challenges posed by AI in finance, potentially leading to new standards and requirements for transparency, accountability, and risk management.
As ai tools in finance become more prevalent, we may also see a shift in the skills and expertise valued in the financial industry, with a greater emphasis on data science, machine learning, and AI development alongside traditional financial knowledge.
Ultimately, the future of AI in investment strategies will depend on how effectively we can harness the power of these technologies while addressing their limitations and ethical implications, to create a more efficient, equitable, and stable financial ecosystem.
Frequently Asked Questions
What AI is used in finance?
AI in finance encompasses a wide range of technologies and applications. Some of the most commonly used AI tools in finance include:
- Machine Learning algorithms for predictive analytics and risk assessment
- Natural Language Processing (NLP) for sentiment analysis and document processing
- Deep Learning neural networks for complex pattern recognition in market data
- Robotic Process Automation (RPA) for streamlining repetitive tasks
- Computer Vision for analyzing visual data like satellite imagery for economic insights
- Reinforcement Learning for developing adaptive trading strategies
These AI technologies are applied across various areas of finance, including investment management, algorithmic trading, fraud detection, customer service, and credit scoring.
Which AI tool is best for financial analysis?
The best AI tool for financial analysis depends on the specific needs and goals of the user. However, some popular AI tools in finance for analysis include:
- Python libraries such as TensorFlow, PyTorch, and scikit-learn for developing custom machine learning models
- IBM Watson for natural language processing and predictive analytics
- Bloomberg’s AI-powered analytics tools for market analysis and risk management
- Alpaca for AI-driven algorithmic trading
- Kensho for AI-powered market intelligence and analytics
- DataRobot for automated machine learning and predictive modeling
It’s important to note that the “best” tool often depends on factors such as the specific use case, data availability, technical expertise, and budget constraints.
How to learn AI tools for finance?
Learning AI tools for finance involves a combination of technical skills and domain knowledge. Here are some steps to get started:
- Develop a strong foundation in mathematics, statistics, and programming (particularly Python or R)
- Take online courses on machine learning and AI, such as those offered by Coursera, edX, or Udacity
- Learn about financial markets, investment strategies, and financial analysis
- Practice implementing AI algorithms on financial datasets using platforms like Kaggle
- Familiarize yourself with popular AI libraries and frameworks used in finance
- Attend workshops, webinars, and conferences focused on AI in finance
- Consider pursuing relevant certifications, such as the Certificate in Quantitative Finance (CQF) or the AI in Finance Certificate from the CFA Institute
- Experiment with open-source AI tools and platforms specifically designed for financial applications
Continuous learning and practical application are key to mastering AI tools in finance.
How is AI used in fintech?
AI is used extensively in fintech to improve efficiency, enhance customer experiences, and develop innovative financial products. Some key applications include:
- Robo-advisors for automated investment management and financial planning
- Fraud detection and prevention systems using machine learning algorithms
- Chatbots and virtual assistants for customer service and support
- Credit scoring models that incorporate alternative data sources
- Algorithmic trading platforms for executing trades at optimal times and prices
- Personalized financial product recommendations based on AI-driven customer profiling
- Risk assessment and management tools for lending and insurance
- Regulatory technology (RegTech) solutions for compliance and reporting
- Blockchain and AI integration for secure and efficient transaction processing
- Predictive analytics for market trends and customer behavior forecasting
These AI applications in fintech are continually evolving, driving innovation and transforming the financial services landscape.
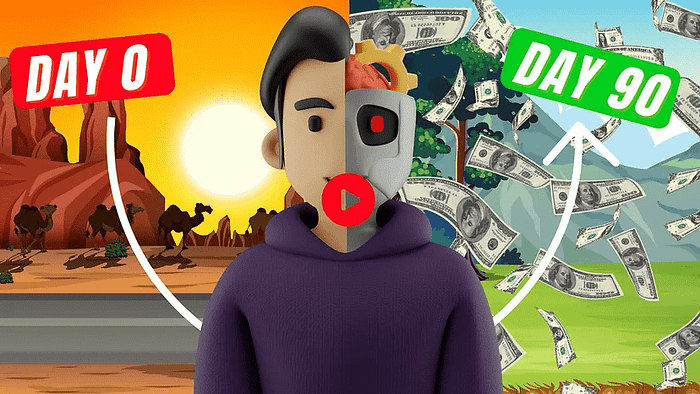
We strongly recommend that you check out our guide on how to take advantage of AI in today’s passive income economy.