Transforming Business Intelligence with AI ROI Metrics
Discovering how to measure AI ROI has become the cornerstone of successful digital transformation, as businesses navigate the complexities of artificial intelligence implementation across various departments.
Understanding the impact of AI investments requires a systematic approach that considers both quantitative and qualitative metrics, ensuring comprehensive evaluation of returns.
Organizations must recognize that measuring AI ROI extends beyond traditional financial metrics, encompassing improved efficiency, enhanced customer satisfaction, and operational excellence.
The journey to effectively measure AI ROI begins with establishing clear baseline metrics before implementation, allowing for accurate comparison and assessment of improvements.
Business leaders who master how to measure AI ROI gain a competitive advantage by optimizing their AI investments and achieving better outcomes across different functions.
Modern enterprises need a structured framework to measure AI ROI, considering the unique challenges and opportunities presented by each business function.
Companies that successfully measure AI ROI can better allocate resources, justify future investments, and drive continuous improvement in their AI initiatives.
Strategic implementation of AI requires careful consideration of both short-term gains and long-term value creation, making it crucial to understand how to measure AI ROI effectively.
We strongly recommend that you check out our guide on how to take advantage of AI in today’s passive income economy.
Table of Contents
Understanding the Fundamentals of AI ROI Measurement
The foundation of learning how to measure AI ROI lies in developing a comprehensive understanding of both direct and indirect benefits that artificial intelligence brings to various business functions.
Organizations must establish clear objectives and key performance indicators (KPIs) specific to each department where AI solutions are implemented.
Success in measuring AI ROI depends on the ability to track and analyze data consistently across different timeframes and business contexts.
Teams need to consider both quantitative metrics like cost savings and revenue growth, as well as qualitative improvements in processes and decision-making.
Effective measurement of AI ROI requires collaboration between technical teams, business analysts, and department heads to ensure accurate data collection and interpretation.
Understanding how to measure AI ROI involves recognizing the unique characteristics of AI implementations and their impact on different business functions.
Companies must develop customized measurement frameworks that align with their specific business goals and industry requirements.
The process of measuring AI ROI should be dynamic and adaptable, evolving as organizations gain more experience with AI implementations.
Sales and Marketing ROI Metrics
When examining how to measure AI ROI in sales and marketing, organizations must focus on key metrics such as lead conversion rates, customer acquisition costs, and revenue growth.
AI implementations in these areas typically show returns through improved targeting, personalized customer experiences, and automated campaign optimization.
Marketing teams can measure AI ROI by tracking improvements in customer engagement, campaign performance, and overall marketing efficiency.
Sales departments benefit from AI through enhanced lead scoring, predictive analytics, and automated customer relationship management.
Organizations learning how to measure AI ROI in sales should monitor metrics like sales cycle length, win rates, and revenue per sales representative.
The impact of AI on customer lifetime value and retention rates provides valuable insights into long-term ROI measurements.
Companies must consider both immediate revenue impacts and long-term customer relationship improvements when measuring AI ROI.
Integration of AI in sales and marketing functions requires careful tracking of both automated and human-assisted interactions for comprehensive ROI assessment.
Operations and Supply Chain Metrics
Measuring AI ROI in operations and supply chain management focuses on efficiency gains, cost reductions, and improved accuracy in forecasting and inventory management.
Organizations must track how AI implementations affect key operational metrics such as inventory turnover, order fulfillment rates, and supply chain visibility.
Understanding how to measure AI ROI in operations requires analysis of both process improvements and resource utilization optimization.
Companies should monitor the impact of AI on reducing operational costs, minimizing errors, and improving overall supply chain resilience.
Implementation of AI in operations often yields measurable improvements in productivity, quality control, and maintenance scheduling.
Organizations must consider the impact of AI on supplier relationships, logistics optimization, and overall operational agility.
Teams should focus on measuring AI ROI through improved forecast accuracy, reduced stockouts, and enhanced supply chain coordination.
The assessment of AI ROI in operations should include both immediate operational improvements and long-term strategic benefits.
Customer Service and Support Metrics
Organizations implementing AI in customer service must understand how to measure AI ROI through metrics like response times, resolution rates, and customer satisfaction scores.
AI-powered customer support systems often demonstrate ROI through reduced handling times, improved first-contact resolution rates, and enhanced customer experiences.
Companies should track both the efficiency gains and quality improvements in customer service delivery when measuring AI ROI.
The impact of AI on customer satisfaction, loyalty, and retention provides valuable insights into the overall return on investment.
Organizations must consider how AI implementations affect both customer-facing metrics and internal operational efficiency in support functions.
Teams learning how to measure AI ROI in customer service should monitor both automated and human-assisted interaction metrics.
The evaluation of AI ROI must include analysis of cost savings, quality improvements, and customer experience enhancements.
Successful measurement of AI ROI in customer service requires consideration of both immediate operational benefits and long-term customer relationship impacts.
Financial and Administrative Metrics
Understanding how to measure AI ROI in financial and administrative functions requires focus on accuracy improvements, processing time reductions, and cost savings.
Organizations must track the impact of AI on key financial metrics such as processing costs, error rates, and compliance adherence.
Companies implementing AI in financial functions should measure improvements in fraud detection, risk assessment, and regulatory compliance.
Administrative processes benefit from AI through automated document processing, improved accuracy, and reduced manual intervention.
Teams must consider both direct cost savings and indirect benefits when learning how to measure AI ROI in administrative functions.
The assessment of AI ROI should include improvements in financial forecasting, budgeting accuracy, and resource allocation efficiency.
Organizations must track both immediate operational improvements and long-term strategic benefits in financial and administrative functions.
Comprehensive measurement of AI ROI requires analysis of both quantitative metrics and qualitative improvements in financial operations.
Human Resources and Talent Management Metrics
Organizations implementing AI in HR functions must understand how to measure AI ROI through improved recruitment efficiency, reduced time-to-hire, and enhanced employee satisfaction.
Companies should track the impact of AI on key HR metrics such as recruitment costs, employee retention rates, and training effectiveness.
The measurement of AI ROI in HR functions must consider both immediate operational improvements and long-term strategic benefits.
Teams must evaluate the impact of AI on employee engagement, productivity, and overall workforce management efficiency.
Organizations learning how to measure AI ROI in HR should monitor both automated and human-driven processes for comprehensive assessment.
The evaluation of AI ROI must include analysis of cost savings, quality improvements, and enhanced decision-making in talent management.
Companies must consider the impact of AI on both operational HR metrics and strategic workforce planning outcomes.
Successful measurement of AI ROI in HR requires consideration of both quantitative improvements and qualitative benefits to organizational culture.
Best Practices and Implementation Strategies
Organizations seeking to maximize AI ROI must develop comprehensive measurement strategies that align with their specific business objectives and industry requirements.
Teams must establish clear baseline metrics before AI implementation to ensure accurate measurement of improvements and returns.
Companies learning how to measure AI ROI should implement regular monitoring and reporting systems to track progress and identify areas for optimization.
The development of standardized measurement frameworks helps ensure consistent evaluation of AI ROI across different business functions.
Organizations must maintain flexibility in their approach to measuring AI ROI, adapting their metrics and methods as needed.
Regular review and refinement of AI ROI measurement strategies help ensure continued alignment with business objectives and market conditions.
Companies should focus on both short-term operational improvements and long-term strategic benefits when measuring AI ROI.
Successful implementation of AI ROI measurement requires ongoing collaboration between technical teams, business analysts, and department heads.
Future Considerations and Scaling AI ROI
Organizations must prepare for evolving requirements in how to measure AI ROI as technology advances and business needs change.
Companies should develop scalable measurement frameworks that can accommodate growth in AI implementations across different functions.
Teams must consider the impact of emerging technologies and changing market conditions on AI ROI measurement strategies.
The future of AI ROI measurement will require increased focus on integration between different business functions and systems.
Organizations must prepare for more sophisticated AI implementations that require advanced measurement techniques and metrics.
Companies learning how to measure AI ROI must stay informed about industry best practices and emerging measurement methodologies.
The evolution of AI technology will create new opportunities and challenges for ROI measurement and optimization.
Successful scaling of AI implementations requires continuous refinement of ROI measurement strategies and frameworks.
Conclusion
Understanding how to measure AI ROI effectively is crucial for organizations seeking to maximize the value of their artificial intelligence investments across different business functions.
Successful measurement of AI ROI requires a balanced approach that considers both quantitative metrics and qualitative improvements.
Organizations must maintain flexibility in their measurement strategies while ensuring consistency in their evaluation methods.
The future of AI ROI measurement will continue to evolve, requiring organizations to adapt and refine their approaches.
Companies that master how to measure AI ROI gain a significant competitive advantage in their digital transformation journey.
Continuous improvement in AI ROI measurement strategies helps ensure optimal returns on artificial intelligence investments.
Organizations must remain focused on both immediate operational benefits and long-term strategic value creation.
The journey to effective AI ROI measurement is ongoing, requiring dedication, adaptation, and continuous learning.
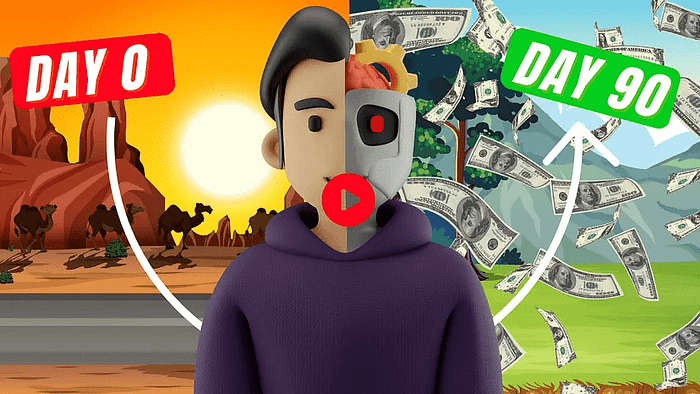
We strongly recommend that you check out our guide on how to take advantage of AI in today’s passive income economy.