Perplexity AI vs. Google: The Future of AI Search Engines
Perplexity AI has transformed how I interact with information online, offering a refreshingly innovative approach to search that stands in stark contrast to traditional engines like Google.
When I first encountered this groundbreaking technology, I was immediately struck by its ability to synthesize information from multiple sources simultaneously.
The difference became apparent within seconds of my initial query—instead of wading through countless blue links, I received a coherent, comprehensive answer that saved valuable time and mental energy.
This revolutionary AI-powered search engine represents a fundamental shift in how we access and process online information, potentially signaling the beginning of Google’s most serious competition in decades.
As digital landscapes evolve rapidly, tools like Perplexity AI, alongside technologies from OpenAI and GPT models, are redefining our expectations for intelligent search capabilities.
The implications extend far beyond simple convenience, potentially restructuring entire information ecosystems and knowledge acquisition methodologies for both casual and professional researchers.
What truly distinguishes Perplexity AI in this emerging battlefield is its ability to understand context, synthesize disparate sources, and present information in ways that feel remarkably human.
Let me take you through my personal journey with this transformative technology and explain why it might represent the future of how we all search for information online.
We strongly recommend that you check out our guide on how to take advantage of AI in today’s passive income economy.
Table of Contents
How Perplexity AI Works
The Technology Behind the Search
Perplexity AI leverages advanced large language models similar to OpenAI’s GPT to process and understand search queries with remarkable nuance and contextual awareness.
Unlike traditional search engines that primarily match keywords and rank websites based on various algorithms, Perplexity conducts multiple simultaneous searches across the internet.
The system then intelligently analyzes, compares, and synthesizes information from numerous sources, effectively doing what would normally require multiple manual searches and cross-referencing.
This approach dramatically reduces the cognitive load typically associated with research, as the AI handles the heavy lifting of information gathering and initial synthesis.
The technology doesn’t simply aggregate information—it comprehends it, restructures it, and presents it in a coherent narrative form that directly addresses your specific query.
Behind the scenes, Perplexity utilizes sophisticated models like GPT-4 Turbo, Claude 3, and Mraw, each bringing unique capabilities to information processing and presentation.
These powerful language models enable Perplexity to understand nuanced questions, recognize implicit needs within queries, and generate responses that feel conversational yet informative.
The integration of these technologies creates a search experience that feels less like using a tool and more like consulting a knowledgeable assistant with instant access to the entire internet.
The User Experience Difference
When I enter a query into Perplexity AI, the response format immediately distinguishes itself from Google’s familiar list of links and snippets.
Instead of presenting multiple options for me to evaluate and click through, Perplexity delivers a comprehensive paragraph that directly addresses my question with synthesized information.
Each statement within this summary includes a footnote reference, allowing me to immediately verify sources and dive deeper into specific aspects that interest me most.
This thoughtful design maintains the critical element of source verification while eliminating the tedious process of jumping between multiple websites to piece together information.
For complex queries like “Tell me the top 10 pizza places near me and why you rank them in that order,” Perplexity provides a complete analysis rather than just listings.
The response includes evaluation criteria, comparative analysis, and supporting evidence—all elements that would typically require multiple searches and significant time investment with traditional engines.
Perhaps most impressively, Perplexity allows users to request reformulations of answers using different underlying AI models, providing varied perspectives on the same information.
This flexibility transforms the search process from passive consumption to active dialogue, creating a dynamic information exchange that adapts to evolving needs and interests.
Practical Applications of Perplexity AI
Research and Information Gathering
When conducting research, Perplexity AI significantly streamlines the information-gathering process through its ability to synthesize data from multiple sources simultaneously.
Traditional research methods often involve hours of searching, reading, comparing, and validating information across numerous websites and publications.
Perplexity compresses this process dramatically by presenting comprehensive summaries with direct source citations, allowing for efficient verification and deeper investigation.
For academic pursuits, this technology enables researchers to quickly establish baseline knowledge on unfamiliar topics before diving into more specialized literature.
The time saved in initial research stages can be redirected toward deeper analysis, critical thinking, and creative problem-solving—the higher-value aspects of intellectual work.
By handling the mechanical aspects of information gathering, Perplexity frees cognitive resources for the uniquely human elements of research: insight generation, pattern recognition, and innovative thinking.
This efficiency gain becomes particularly valuable when exploring interdisciplinary topics that traditionally require navigating multiple knowledge domains and terminologies.
The technology essentially democratizes research capabilities, providing individual users with information synthesis powers previously available only to those with research teams or extensive experience.
News and Current Events
Perplexity AI excels at providing balanced, comprehensive overviews of current events by aggregating information from multiple news sources and presenting synthesized summaries.
When I ask about today’s headlines, the system searches numerous news outlets, identifying common threads, unique perspectives, and consensus viewpoints across the media landscape.
This approach helps mitigate the filter bubble effect common to algorithm-driven news consumption, exposing users to diverse perspectives on complex issues.
The resulting summaries include proper attribution through footnotes, enabling me to investigate original sources when I want to explore particular viewpoints or reporting approaches.
For time-sensitive information, this aggregation capability proves invaluable, providing rapid situational awareness without requiring manual monitoring of multiple news platforms.
The technology effectively serves as a personalized news briefing service, adapting to specific interests while maintaining breadth of coverage and source diversity.
When integrated with technologies like OpenAI’s GPT, these capabilities become even more sophisticated, offering nuanced analysis of evolving situations across global contexts.
This represents a significant evolution in information consumption, moving beyond both traditional news models and first-generation algorithmic news aggregators toward truly intelligent synthesis.
Travel Planning and Decision Making
Planning trips traditionally requires visiting numerous websites—airlines, hotels, review sites, travel blogs, and local guides—to compile comprehensive information.
Perplexity AI transforms this process by simultaneously searching multiple travel resources and synthesizing the information into coherent, actionable recommendations.
A single query like “Plan a three-day trip to Barcelona with cultural highlights and local cuisine” yields a comprehensive itinerary with options, pricing information, and insider tips.
Each recommendation includes source citations, allowing me to verify details, read reviews, or explore alternatives without starting additional search processes from scratch.
The system intelligently balances popular attractions with less-known options, high and low-cost alternatives, and diverse experiences based on aggregated recommendations.
This approach saves hours of planning time while potentially introducing options I might never have discovered through traditional search methods.
For decision-making processes involving multiple variables and preferences, Perplexity excels at presenting balanced information that acknowledges tradeoffs and alternatives.
The ability to follow up with clarifying questions further enhances the planning process, creating an interactive experience more akin to consulting a knowledgeable travel agent than using a search engine.
Comparing Perplexity AI and Google
Search Methodology Differences
Google’s traditional search methodology relies primarily on matching keywords, evaluating page authority, and ranking results based on complex algorithms including user engagement metrics.
Perplexity AI fundamentally reimagines this approach by focusing first on understanding the query intent and then actively searching for and synthesizing relevant information.
While Google provides options for users to evaluate and select from, Perplexity takes on the cognitive load of evaluation and synthesis, presenting conclusions rather than possibilities.
Google excels at providing access to specific websites and diverse information sources but requires users to manually compile and compare information across multiple pages.
Perplexity prioritizes efficiency and comprehensiveness, focusing on delivering complete answers rather than pathways to potential information sources.
The difference becomes particularly apparent with complex queries requiring information from multiple domains or involving comparative analysis.
Where Google might return results from several categories—product listings, review sites, forums, and guides—Perplexity integrates these perspectives into a unified response.
This fundamental difference reflects evolving user expectations, increasingly prioritizing direct answers over resource discovery in many search contexts.
User Interface and Experience
The visual presentation of Perplexity responses bears little resemblance to Google’s familiar blue links, snippets, and various SERP features accumulated over decades.
Instead of a vertically scrolling list of potential resources, Perplexity presents a conversational interface centered around comprehensive text responses.
Each response paragraph includes numerically sequenced footnotes, creating an academic-style reference system that maintains intellectual rigor within a conversational format.
Clicking these footnotes immediately reveals source information, maintaining transparency while streamlining the verification process compared to traditional search.
The interface prioritizes readability and information density, eliminating distractions like advertisements that often fragment attention in traditional search environments.
Perplexity’s design encourages deeper engagement with content rather than rapid scanning and clicking, potentially improving information retention and understanding.
The ability to request reformulations using different AI models introduces an interactive dimension absent from traditional search, creating dialogue rather than discrete searches.
This conversational approach more naturally accommodates the iterative nature of genuine inquiry, where initial questions evolve based on progressive understanding.
Accuracy and Source Verification
Concerns about AI hallucinations and factual accuracy represent significant challenges for AI-powered search tools like Perplexity compared to traditional engines.
Perplexity addresses these concerns through its footnote citation system, providing immediate source verification for every claim presented in its summaries.
This approach combines the efficiency of AI synthesis with the reliability of traditional source-based research methodologies.
When the system encounters contradictory information across sources, it typically acknowledges these discrepancies rather than arbitrarily selecting a single perspective.
For factual queries, this transparency helps users evaluate the reliability of information based on source credibility and consensus across multiple references.
The system remains vulnerable to inaccuracies present in source material, but by exposing these sources directly, it provides users with tools for critical evaluation.
Integration with technologies from OpenAI and other leading AI developers continues to improve factual accuracy and reduce instances of synthesized misinformation.
This hybrid approach—AI synthesis with transparent source attribution—represents a promising evolution in balancing efficiency with accuracy in information retrieval.
Real-World Applications and Limitations
Professional Use Cases
In professional contexts, Perplexity AI demonstrates particular value for roles requiring rapid information synthesis across multiple knowledge domains.
Journalists researching breaking stories can quickly establish factual foundations while identifying diverse perspectives and potential contradictions for further investigation.
Legal professionals can efficiently survey case precedents and relevant statutes across jurisdictions before conducting deeper analysis of specific applicable cases.
Healthcare providers can stay updated on emerging treatment approaches and research findings across specialties without manually monitoring numerous medical journals.
Marketing strategists can rapidly assess competitive landscapes, consumer sentiment trends, and regulatory considerations when developing campaign approaches.
Financial analysts can compile market reactions, expert opinions, and historical contexts when evaluating investment opportunities or economic developments.
Project managers can efficiently gather technical specifications, regulatory requirements, and implementation best practices when planning complex initiatives.
Educational professionals can quickly compile diverse teaching approaches, learning resources, and assessment methodologies when developing curriculum materials.
Current Limitations
Despite its impressive capabilities, Perplexity AI currently faces several limitations that affect its utility in certain contexts compared to established search engines.
The system occasionally struggles with highly technical or specialized queries where relevant information exists primarily in academic databases or specialized professional resources.
For some local queries, particularly in less-populated areas, traditional search engines may still provide more comprehensive results due to their established indexing systems.
Perplexity’s synthesis capabilities, while advanced, sometimes oversimplify complex topics where nuance and disciplinary context are essential for accurate understanding.
The system remains dependent on the quality and currency of its source material, occasionally incorporating outdated information when more recent updates are available.
For some specialized professional applications, integration with proprietary databases and information systems remains limited compared to enterprise search solutions.
Multimedia search capabilities, particularly for images, videos, and audio content, currently lag behind the sophisticated options available through established search platforms.
Language support beyond English, while improving, has not yet reached parity with major search engines’ capabilities across global languages and dialectal variations.
The Future of AI Search
Potential Developments
As AI search technologies mature, we can anticipate several transformative developments that will further differentiate tools like Perplexity from traditional search engines.
Multimodal capabilities will likely expand, enabling searches that seamlessly integrate text, image, audio, and video understanding for more comprehensive information retrieval.
Personalization algorithms will evolve to balance individual relevance with exposure to diverse perspectives, potentially addressing filter bubble concerns more effectively than current systems.
Real-time knowledge integration will accelerate, reducing the gap between information publication and its incorporation into AI search responses.
Domain-specific capabilities will emerge, with specialized AI search tools optimized for fields like medicine, law, engineering, and academic research.
Interactive visualization features will enhance data-heavy responses, automatically generating charts, graphs, and other visual representations to improve comprehension.
Collaborative search capabilities will develop, enabling multiple users to jointly explore topics with AI assistance for team research projects.
Integration with productivity tools will deepen, allowing seamless transitions from information discovery to application in documents, presentations, and decision-making frameworks.
These developments, building on frameworks established by OpenAI, GPT, and other foundational AI technologies, will continue reshaping our relationship with online information.
Implications for Traditional Search
Google and other traditional search engines face significant strategic decisions as AI search alternatives gain traction among both casual and professional users.
The fundamental value proposition of search is evolving from providing access to information toward delivering understanding and actionable insights.
This shift challenges core business models built around search advertising, as AI-synthesized responses potentially reduce exposure to traditional ad placements.
We may witness hybrid approaches emerging, with traditional search engines incorporating more AI synthesis while maintaining their strengths in comprehensive indexing.
The competitive landscape will likely drive rapid innovation cycles, benefiting users through accelerated feature development and performance improvements.
Enterprise and specialized professional search markets may segment from general consumer search, with different solutions optimized for different use contexts.
Privacy considerations will gain prominence as AI search capabilities increasingly depend on understanding user context and history for maximum relevance.
The ultimate outcome may not be winner-take-all but rather an ecosystem of complementary search approaches optimized for different information needs and contexts.
Conclusion
My transition from Google to Perplexity AI represents more than a simple tool switch—it signals a fundamental evolution in how we interact with the vast information landscape online.
The traditional search paradigm, revolutionary in its time, increasingly feels inefficient when compared to AI-powered alternatives that handle not just discovery but synthesis.
As these technologies continue to mature, incorporating advances from OpenAI, GPT, and other AI research leaders, the gap between traditional and AI-enhanced search will likely widen.
The most significant impact may be measured not in technical metrics but in time reclaimed—hours previously spent manually compiling and comparing information now available for deeper thinking.
For casual users, this efficiency translates to more informed daily decisions without prohibitive research investments.
For professionals, it enables more time focused on unique human contributions: creativity, critical analysis, and innovative problem-solving.
The future search landscape will likely feature multiple specialized tools rather than universal solutions, with users selecting approaches based on specific information needs.
As we navigate this transition, maintaining healthy skepticism while embracing genuine innovation will help us harness these powerful tools while mitigating potential risks in our evolving relationship with AI-mediated information.
Additional Resources
Getting Started with Perplexity AI
For those interested in experiencing Perplexity AI firsthand, the platform offers both free access and premium subscription options with enhanced capabilities.
New users can begin with simple factual queries before progressing to more complex research questions that demonstrate the system’s synthesis capabilities.
Experimenting with different AI models (GPT-4 Turbo, Claude 3, etc.) for response generation provides insight into the nuances of various approaches to information synthesis.
The platform works effectively across devices, with dedicated mobile applications providing on-the-go access to advanced search capabilities.
Learning to formulate effective queries represents the primary learning curve—questions that clearly specify information needs and evaluation criteria yield the best results.
Regular users may discover productivity benefits from integrating Perplexity into research workflows, particularly for initial topic exploration and comprehensive overviews.
Educators and students might find particular value in the platform’s ability to provide balanced information with clear source attribution for learning projects.
Professional researchers can leverage the technology as a complementary tool alongside specialized databases and traditional search methods for comprehensive information gathering.
Understanding AI Search Limitations
As we embrace these powerful tools, maintaining awareness of their limitations helps establish appropriate usage contexts and expectation management.
All AI search tools, including Perplexity, remain dependent on their training data and the quality of information available online about any given topic.
Expertise in critically evaluating source quality remains essential, as AI systems may not fully account for authoritativeness or specialized credibility indicators.
Some information needs—particularly those requiring deep domain expertise or access to proprietary data—may still be better served through specialized channels.
Cultural and linguistic nuances sometimes present challenges for AI systems trained predominantly on English-language content from certain cultural contexts.
Privacy considerations warrant attention, as comprehensive search assistance potentially requires access to personal context information for maximum relevance.
The rapidly evolving nature of these technologies means capabilities and limitations shift continuously, requiring ongoing adaptation in usage approaches.
Maintaining healthy skepticism while exploring the genuine benefits of these tools represents the most balanced approach to integrating AI search into information workflows.
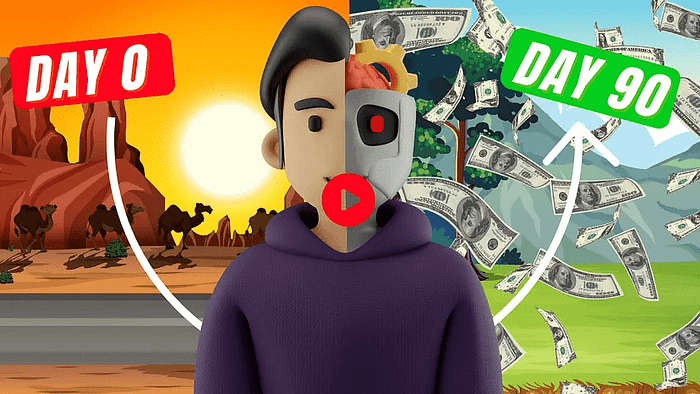
We strongly recommend that you check out our guide on how to take advantage of AI in today’s passive income economy.