How Multi-head Latent Attention is Revolutionizing AI Memory Efficiency
Groundbreaking innovations in artificial intelligence often emerge from unexpected places, and the revolutionary Multi-head Latent Attention (MLA) technology represents a pivotal moment in AI development. This remarkable advancement in memory efficiency is transforming how we approach large language models and their computational requirements, making advanced AI more accessible and sustainable than ever before.
We strongly recommend that you check out our guide on how to take advantage of AI in today’s passive income economy.
Table of Contents
The Memory Challenge in Modern AI
The computational demands of running advanced AI models have long been a significant barrier to widespread adoption and development. Traditional transformer models, which power modern language models like GPT and Claude, utilize Multi-Head Attention (MHA) mechanisms that require enormous amounts of memory. This memory-intensive process becomes increasingly challenging as conversations and text processing requirements grow longer.
In the current landscape of AI development, the memory requirements for processing each token in traditional transformer models are staggering. With standard MHA, each token demands 8192 KB of cache memory, creating a substantial computational burden that limits accessibility and scalability. This challenge has been a persistent obstacle in democratizing AI technology and expanding its applications across various sectors.
Understanding the Deep Sea Breakthrough
DeepSeek, a Chinese AI research company, has emerged as a pioneering force in addressing this fundamental challenge. Their innovative approach to memory efficiency has resulted in a breakthrough that dramatically reduces memory usage while maintaining performance standards. The development of Multi-head Latent Attention represents a paradigm shift in how AI models handle and process information.
The Technical Innovation Behind MLA
To truly appreciate the significance of MLA, we must understand its foundational principles. The technology functions similarly to an extremely efficient library cataloging system, where information is compressed and organized in a way that maximizes accessibility while minimizing storage requirements. This innovative approach to data management has resulted in an astounding 93.3% reduction in memory usage compared to traditional methods.
Breaking Down the Technology
The core of MLA’s efficiency lies in its sophisticated compression technique, which utilizes low-rank approximation to compress key and value matrices during the training process. This integration of compression during training, rather than as a post-processing step, is crucial for maintaining optimal performance. The system employs multiple Lo L functions to ensure quality and consistency in data processing.
Real-World Impact and Performance Metrics
The practical implications of this breakthrough are substantial and far-reaching. Deep Sea’s implementation of MLA has demonstrated remarkable improvements across multiple performance metrics. Beyond the 93.3% reduction in memory usage, the technology has achieved a 5.76x improvement in generation speed and a 42.5% reduction in training costs.
Democratizing AI Access
This significant reduction in computational requirements means that AI tasks that previously required high-end hardware and specialized chips can now run on more modest systems. The analogy to Apple’s revolutionary “thousand songs in your pocket” moment is apt, as MLA similarly transforms how we think about AI accessibility and portability.
Understanding the Limitations and Future Potential
While MLA represents a significant breakthrough, it’s important to acknowledge its current limitations. Research indicates slightly higher perplexity in smaller models and some additional computational overhead from the compression process. However, these limitations are minimal compared to the technology’s benefits and potential for future development.
The Innovation Approach
DeepSeek’s success in developing MLA challenges traditional approaches to AI research. Rather than relying solely on experienced experts in transformer models, the company recruited university students and fresh thinkers who could approach problems without preconceived notions. This strategy of embracing new perspectives has proven instrumental in achieving breakthrough innovations.
Broader Implications for AI Development
The impact of MLA extends far beyond technical specifications. This breakthrough has significant implications for AI accessibility, sustainability, and future development potential. The dramatic reduction in memory requirements opens new possibilities for longer context windows and more advanced reasoning capabilities in AI models.
Environmental and Economic Benefits
The efficiency gains from MLA technology have important environmental implications. Reduced memory requirements mean less energy consumption and potentially fewer data centers needed for AI operations. This improvement in sustainability aligns with growing concerns about the environmental impact of AI development and deployment.
Future Prospects and Industry Response
The introduction of MLA technology has sparked considerable interest in how major AI companies might implement and build upon this breakthrough. With companies like OpenAI potentially applying these techniques with their substantial resources, we could see even more powerful and efficient AI models in the future.
Implications for AI Accessibility
The reduced memory requirements and improved efficiency of MLA technology have significant implications for democratizing AI access. This breakthrough could enable broader implementation of advanced AI capabilities across various sectors and applications, potentially transforming how we interact with and utilize AI technology.
Looking Ahead: The Future of AI Memory Efficiency
The development of MLA technology represents a crucial moment in AI innovation history. As the technology continues to evolve and improve, we may see even more dramatic improvements in efficiency and capability. The potential for longer context windows and more sophisticated reasoning capabilities could unlock new applications and possibilities in AI development.
Conclusion
The breakthrough in Multi-head Latent Attention technology marks a significant milestone in AI development, promising more efficient, accessible, and sustainable AI systems. As this technology continues to evolve and be adopted across the industry, we may be witnessing the beginning of a new era in artificial intelligence, where advanced AI capabilities become more accessible to researchers, developers, and users worldwide.
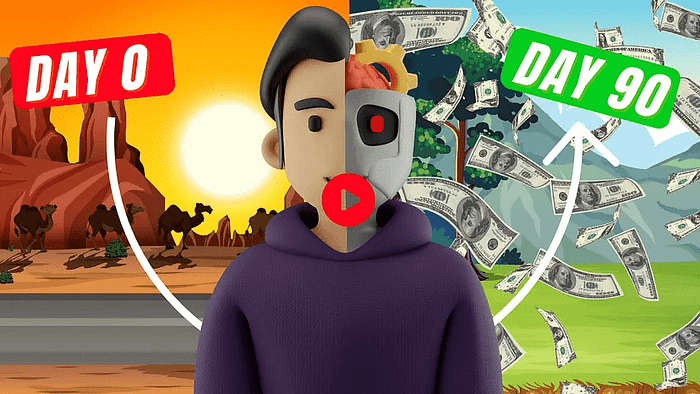
We strongly recommend that you check out our guide on how to take advantage of AI in today’s passive income economy.