The Next Big Thing: AI Agent Technology Explained and Demystified
The dawn of AI agent technology explained heralds a new era in artificial intelligence, reshaping our digital landscape.
As someone deeply immersed in the world of technology, I’ve witnessed firsthand the rapid evolution of AI agents.
These intelligent entities are far more than just lines of code; they’re the harbingers of a technological revolution that’s set to transform every aspect of our lives.
In this article, I’ll take you on a journey through the fascinating world of AI agents, exploring their capabilities, types, and the profound impact they’re poised to have on our future.
So, buckle up and get ready to dive into the exciting realm of AI agent technology explained!
We strongly recommend that you check out our guide on how to take advantage of AI in today’s passive income economy.
Table of Contents
What Are AI Agents?
AI agents are sophisticated software entities designed to interact with and navigate their environment autonomously.
Unlike traditional programs, these agents possess the ability to sense, process, and act upon information from their surroundings.
This capability allows them to make decisions and take actions independently, often mimicking human-like intelligence.
AI agent technology explained encompasses a wide range of applications, from simple task-oriented programs to complex decision-making systems.
These agents are the building blocks of advanced artificial intelligence systems, capable of learning, adapting, and evolving over time.
As we delve deeper into AI agent technology explained, we’ll uncover the intricate workings of these digital marvels.
The Core Components of AI Agents
At the heart of AI agent technology explained lies a trio of essential components: sensors, processors, and actuators.
Sensors act as the agent’s eyes and ears, collecting data from the environment.
This could be anything from user inputs to complex sensor arrays in robotics.
The processors are the brain of the agent, analyzing the collected data and making decisions based on predefined algorithms or learned behaviors.
Actuators are the means by which the agent interacts with its environment, executing the decisions made by the processor.
This could be as simple as displaying text on a screen or as complex as controlling robotic limbs.
Together, these components form the foundation of AI agent technology explained, enabling agents to perceive, think, and act in their digital or physical worlds.
The Evolution of AI Agents
The journey of AI agent technology explained is a fascinating tale of technological progress.
From the early days of simple rule-based systems to today’s sophisticated machine learning models, AI agents have come a long way.
The advent of deep learning and neural networks has revolutionized AI agent technology explained, enabling agents to learn from vast amounts of data and improve their performance over time.
This evolution has led to AI agents that can understand natural language, recognize images, and even engage in complex reasoning tasks.
As we continue to push the boundaries of AI agent technology explained, we’re witnessing the birth of agents that can rival human intelligence in specific domains.
The future promises even more exciting developments in AI agent technology explained, with agents becoming increasingly autonomous and capable of handling complex, real-world tasks.
Types of AI Agents
Simple Reflex Agents
Simple reflex agents represent the most basic form of AI agent technology explained.
These agents operate on a straightforward principle: they perceive their environment and react based on predefined rules.
Imagine a thermostat that turns on the heating when the temperature drops below a certain point.
This is a classic example of a simple reflex agent in action.
While their decision-making process is elementary, these agents are incredibly efficient for tasks that require immediate, rule-based responses.
In the realm of AI agent technology explained, simple reflex agents serve as the foundation upon which more complex agents are built.
Their simplicity makes them reliable and predictable, ideal for scenarios where quick, consistent responses are crucial.
However, their inability to consider past experiences or predict future outcomes limits their applicability in more complex environments.
Model-Based Reflex Agents
Model-based reflex agents represent a step up in AI agent technology explained.
These agents maintain an internal model of their environment, allowing them to make decisions based on both current perceptions and their understanding of how the world works.
This internal model enables them to handle partially observable environments more effectively than simple reflex agents.
For instance, a self-driving car uses a model-based approach to navigate, considering not just what it can see but also what it knows about road rules and typical traffic patterns.
In the context of AI agent technology explained, model-based agents demonstrate the power of combining immediate perception with stored knowledge.
This combination allows them to make more informed decisions, even when they don’t have complete information about their environment.
The ability to maintain and update an internal model makes these agents more adaptable and capable of handling a wider range of scenarios.
Goal-Based Agents
Goal-based agents embody a more advanced form of AI agent technology explained.
These agents are designed with specific objectives in mind and can plan and execute actions to achieve these goals.
Unlike reflex agents, goal-based agents consider the future consequences of their actions.
They evaluate different courses of action and choose the one most likely to lead to their desired outcome.
For example, a chess-playing AI agent doesn’t just react to the current board state; it plans several moves ahead to achieve its goal of winning the game.
In the realm of AI agent technology explained, goal-based agents represent a significant leap forward in decision-making capabilities.
They can handle complex, multi-step problems and adapt their strategies as circumstances change.
This goal-oriented approach makes them ideal for tasks that require long-term planning and strategic thinking.
Utility-Based Agents
Utility-based agents take AI agent technology explained to an even higher level of sophistication.
These agents don’t just work towards a goal; they aim to maximize a specific utility function.
This function assigns a value to different states or outcomes, allowing the agent to make decisions based on what it considers most beneficial.
A utility-based agent in a financial trading system, for instance, might make decisions not just to profit, but to balance profit with risk according to a predefined utility function.
In the landscape of AI agent technology explained, utility-based agents shine in scenarios where there are multiple competing goals or where the best outcome isn’t always clear-cut.
They can handle complex trade-offs and make nuanced decisions based on a comprehensive evaluation of potential outcomes.
This makes them particularly valuable in fields like economics, resource management, and strategic planning.
Learning Agents
Learning agents represent the cutting edge of AI agent technology explained.
These agents have the remarkable ability to improve their performance over time through experience.
They start with a basic set of knowledge or rules and then refine and expand this knowledge as they interact with their environment.
Machine learning algorithms power these agents, enabling them to recognize patterns, make predictions, and adapt to new situations.
A prime example of learning agents in action is in recommendation systems that improve their suggestions based on user feedback.
In the realm of AI agent technology explained, learning agents are perhaps the most exciting development.
Their ability to evolve and improve autonomously opens up endless possibilities for AI applications.
From personalized assistants that understand our preferences to adaptive control systems in complex industrial processes, learning agents are pushing the boundaries of what’s possible in AI.
Multi-Agent Systems
Multi-agent systems (MAS) represent a fascinating aspect of AI agent technology explained.
These systems consist of multiple agents working together or competing to solve problems or achieve goals.
Each agent in the system may have its own objectives, knowledge, and capabilities, but they interact within a shared environment.
This interaction can lead to emergent behaviors that are more complex and sophisticated than what any single agent could achieve alone.
For instance, in a traffic management system, multiple AI agents might represent different intersections, working together to optimize traffic flow across an entire city.
In the context of AI agent technology explained, multi-agent systems demonstrate the power of collective intelligence.
They can tackle complex, distributed problems that would be difficult or impossible for a single agent to handle.
MAS are particularly valuable in scenarios like supply chain management, disaster response coordination, and collaborative robotics.
Hierarchical Agents
Hierarchical agents represent a structured approach to AI agent technology explained.
These agents are organized in a hierarchical manner, with higher-level agents overseeing and coordinating the actions of lower-level agents.
This structure allows for the decomposition of complex tasks into simpler subtasks, each handled by a specialized agent.
The hierarchical organization enables efficient decision-making and task execution across different levels of abstraction.
A robotic system in a manufacturing plant might use a hierarchical agent structure, with high-level agents planning overall production strategies and lower-level agents controlling individual machine operations.
In the landscape of AI agent technology explained, hierarchical agents offer a powerful way to manage complexity in large-scale systems.
They allow for the integration of different types of agents, each optimized for specific tasks, into a cohesive whole.
This approach is particularly valuable in scenarios that require both high-level strategic planning and detailed operational control.
The Impact of AI Agents on Various Industries
AI Agents in Healthcare
The integration of AI agent technology explained in healthcare is revolutionizing patient care and medical research.
AI agents are being deployed to analyze medical images, assist in diagnosis, and even predict potential health issues before they become serious.
These agents can process vast amounts of medical data, identifying patterns and insights that might escape even the most experienced human practitioners.
For instance, AI agents are now capable of detecting early signs of diseases like cancer in medical scans with remarkable accuracy.
In the realm of AI agent technology explained, healthcare applications demonstrate the life-saving potential of these intelligent systems.
From personalized treatment plans to drug discovery, AI agents are accelerating medical advancements and improving patient outcomes.
As AI agent technology explained continues to evolve, we can expect even more groundbreaking applications in healthcare, potentially transforming the way we approach medicine and wellness.
AI Agents in Finance
The financial sector has been quick to embrace AI agent technology explained, leveraging these intelligent systems to enhance decision-making and risk management.
AI agents are now integral to many aspects of finance, from algorithmic trading to fraud detection and credit scoring.
These agents can analyze market trends, predict price movements, and execute trades at speeds far beyond human capabilities.
In personal finance, AI agents are powering robo-advisors that provide automated, personalized investment advice to individual investors.
The application of AI agent technology explained in finance showcases the power of data-driven decision-making.
These agents can process enormous volumes of financial data in real-time, identifying opportunities and risks that human analysts might miss.
As AI agent technology explained continues to advance, we can expect even more sophisticated financial applications, potentially reshaping the entire landscape of global finance.
AI Agents in Manufacturing
The manufacturing sector is undergoing a transformation thanks to AI agent technology explained.
Intelligent agents are being deployed across the entire manufacturing process, from design and planning to production and quality control.
These agents can optimize production schedules, predict equipment failures before they occur, and ensure consistent product quality.
In smart factories, AI agents control robotic systems, coordinate supply chains, and manage energy consumption, leading to unprecedented levels of efficiency and productivity.
The impact of AI agent technology explained in manufacturing extends beyond the factory floor.
These agents are enabling new paradigms like mass customization, where products can be tailored to individual customer preferences without sacrificing production efficiency.
As AI agent technology explained continues to evolve, we can expect to see even more innovative applications in manufacturing, potentially ushering in a new era of industrial production.
AI Agents in Transportation
The transportation industry is being reshaped by AI agent technology explained, with applications ranging from self-driving vehicles to intelligent traffic management systems.
AI agents are at the heart of autonomous vehicle technology, processing sensor data, making split-second decisions, and navigating complex road environments.
In public transportation, AI agents optimize routes, predict maintenance needs, and improve overall system efficiency.
For logistics and shipping, AI agents are revolutionizing supply chain management, route planning, and last-mile delivery.
The application of AI agent technology explained in transportation showcases the potential for increased safety, efficiency, and sustainability in how we move people and goods.
These intelligent systems can reduce traffic congestion, lower emissions, and potentially eliminate human error in driving.
As AI agent technology explained continues to advance, we can expect to see even more transformative applications in transportation, potentially reshaping our cities and how we think about mobility.
The Future of AI Agents
Ethical Considerations
As AI agent technology explained continues to advance, we must grapple with the ethical implications of these intelligent systems.
The increasing autonomy and decision-making capabilities of AI agents raise important questions about responsibility, accountability, and the potential for unintended consequences.
We need to consider how to ensure that AI agents act in ways that align with human values and societal norms.
This includes addressing issues of bias in AI decision-making, protecting privacy, and ensuring transparency in how AI agents reach their conclusions.
As we continue to develop AI agent technology explained, it’s crucial that we also develop robust ethical frameworks and governance structures.
We need to strike a balance between harnessing the potential of AI agents and safeguarding against potential risks.
This will require ongoing dialogue between technologists, ethicists, policymakers, and the public to shape the future of AI in a responsible and beneficial way.
Integration with Emerging Technologies
The future of AI agent technology explained is closely intertwined with other emerging technologies.
The integration of AI agents with technologies like the Internet of Things (IoT), blockchain, and quantum computing promises to unlock new realms of possibility.
For instance, AI agents combined with IoT devices could create incredibly responsive and efficient smart cities.
AI agents leveraging blockchain technology could enable new forms of secure, decentralized decision-making systems.
The combination of AI agent technology explained with quantum computing could lead to unprecedented advances in problem-solving capabilities.
These integrated systems could tackle complex global challenges like climate change, resource management, and disease control with a level of sophistication we can barely imagine today.
As these technologies converge, we can expect to see AI agents that are more powerful, more interconnected, and more deeply embedded in every aspect of our lives.
Human-AI Collaboration
The future of AI agent technology explained isn’t about replacing humans, but rather about enhancing human capabilities.
We’re moving towards a future where AI agents and humans work together in symbiotic relationships, each complementing the strengths of the other.
AI agents can handle vast amounts of data and complex calculations, while humans provide creativity, emotional intelligence, and ethical judgment.
This collaboration is already happening in fields like scientific research, where AI agents assist human scientists in analyzing data and generating hypotheses.
As AI agent technology explained continues to advance, we can expect to see even more sophisticated forms of human-AI collaboration.
This could lead to breakthroughs in fields ranging from medicine to space exploration, pushing the boundaries of what’s possible when human ingenuity is amplified by artificial intelligence.
The key to this future will be developing AI systems that are not just intelligent, but also intuitive and easy for humans to work with.
Conclusion
As we’ve explored throughout this article, AI agent technology explained represents a paradigm shift in how we interact with and leverage artificial intelligence.
From simple reflex agents to complex learning systems, these digital entities are reshaping industries, solving complex problems, and opening up new possibilities for innovation.
The future of AI agents is bright, filled with potential for groundbreaking advancements across every sector of society.
However, as we continue to develop and deploy these powerful tools, we must also navigate the ethical considerations and societal implications that come with them.
The rise of AI agents isn’t just a technological revolution; it’s a transformation of how we live, work, and think.
As we stand on the brink of this new era, one thing is clear: AI agent technology explained will play a crucial role in shaping our future.
It’s up to us to guide this technology responsibly, ensuring that it serves to enhance human capabilities and improve lives around the globe.
The journey of AI agent technology explained is just beginning, and the most exciting chapters are yet to be written.
Frequently Asked Questions:
How does the AI agent work?
AI agent technology explained revolves around a three-step process: perception, reasoning, and action.
The agent first perceives its environment through sensors or data inputs.
This information is then processed using algorithms or machine learning models to reason about the best course of action.
Finally, the agent acts on its decision through various outputs or actuators.
For example, a self-driving car agent perceives its surroundings through cameras and sensors, reasons about traffic rules and obstacles, and then acts by controlling the car’s steering, acceleration, and braking.
The specifics of how an AI agent works can vary depending on its type and purpose, but this basic cycle of perceive-reason-act is fundamental to AI agent technology explained.
What are the 5 types of agents in AI?
AI agent technology explained encompasses various types of agents, but five common types are:
- Simple Reflex Agents: These agents act based on the current perception of their environment, using predefined rules.
- Model-Based Reflex Agents: These maintain an internal model of their world, allowing them to handle partially observable environments.
- Goal-Based Agents: These agents work towards achieving specific goals, planning their actions accordingly.
- Utility-Based Agents: These make decisions based on a utility function that measures the desirability of different outcomes.
- Learning Agents: These can improve their performance over time through experience, adapting to new situations.
Each type of agent in AI agent technology explained has its strengths and is suited for different types of tasks and environments.
How to implement an AI agent?
Implementing an AI agent involves several steps:
- Define the problem: Clearly outline what the agent needs to achieve.
- Choose the agent type: Based on the problem, select the most appropriate type of agent.
- Design the environment: Define how the agent will interact with its surroundings.
- Implement the agent’s components: This includes sensors (for input), actuators (for output), and the core decision-making logic.
- Develop the agent’s knowledge base: This could be rules, models, or training data, depending on the agent type.
- Implement the learning mechanism: For learning agents, design how they will improve over time.
- Test and refine: Evaluate the agent’s performance and iterate on the design.
AI agent technology explained emphasizes the importance of careful planning and design in implementation.
The specific implementation details can vary greatly depending on the complexity of the agent and the problem it’s solving.
What is the AI agent framework?
An AI agent framework is a software toolkit that provides the necessary components and structures for developing AI agents.
These frameworks offer pre-built modules for common agent functionalities, allowing developers to focus on the specific logic of their agent.
Key components of an AI agent framework typically include:
- Environment simulators
- Sensor and actuator interfaces
- Decision-making modules
- Learning algorithms
- Knowledge representation structures
- Communication protocols for multi-agent systems
Popular AI agent frameworks include OpenAI Gym for reinforcement learning, JADE for multi-agent systems, and TensorFlow Agents for building intelligent agents.
AI agent technology explained highlights the importance of these frameworks in streamlining the development process and enabling the creation of more complex and capable agents.
These frameworks continue to evolve, incorporating new advances in AI agent technology explained to provide developers with powerful tools for creating intelligent systems.
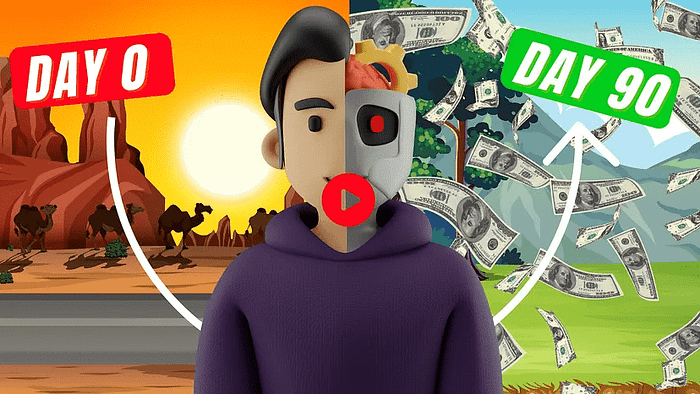
We strongly recommend that you check out our guide on how to take advantage of AI in today’s passive income economy.