I Dove Deep into Google Agent Builder: Here’s What You Need to Know
Google’s Agent Builder burst onto the scene, promising to revolutionize AI agent development.
As a curious AI enthusiast, I couldn’t resist diving in to explore this new tool.
What I discovered was both fascinating and frustrating.
In this comprehensive review, I’ll take you through my journey with Google Agent Builder, sharing insights that could save you time and potential headaches.
From its enterprise focus to its surprising limitations, we’ll uncover the truth behind this much-hyped platform.
So, buckle up as we embark on this eye-opening exploration of Google’s latest AI offering.
We strongly recommend that you check out our guide on how to take advantage of AI in today’s passive income economy.
Table of Contents
Accessing Google Agent Builder
The Labyrinthine Path to Agent Creation
My adventure with Google Agent Builder began with an unexpected challenge: simply accessing the tool.
The process felt like navigating a digital maze, far from the seamless experience I anticipated.
To start, I had to log into Google Cloud, which seemed reasonable enough.
However, the real confusion set in when I tried to locate the Agent Builder within the vast ecosystem of Google’s cloud services.
After some trial and error, I discovered that the path to Agent Builder was hidden under “Apps” and then “Chat, recommendations, and agent” in the bottom right corner.
This convoluted route made me wonder about the user-friendliness of the platform, especially for those less familiar with Google’s cloud infrastructure.
Regional Restrictions and URL Oddities
As I delved deeper into the setup process, I encountered an unexpected hurdle: regional restrictions.
Initially, I attempted to create an agent using the “Global” region option, as I’m not based in the US.
To my surprise, this selection was met with an error message, forcing me to switch to the US region to proceed.
This limitation seemed at odds with Google’s global presence and could potentially alienate users from other parts of the world.
Adding to the peculiarity of the experience was the URL structure for Google Agent Builder.
The address bar displayed “Tex AIC conversation.cloud.google.com”, which struck me as unusually complex and potentially confusing.
Such an unconventional URL could easily be mistaken for a phishing attempt, raising concerns about user trust and security perception.
Building Your First Agent with Google Agent Builder
Setting Up the Agent’s Foundation
With access finally granted, I eagerly began the process of creating my first AI agent using Google Agent Builder.
The interface presented a split-screen layout, reminiscent of other AI development platforms.
On the left side, I found the tools for building and configuring the agent, while the right side served as a testing ground.
This familiar structure provided a sense of comfort, suggesting that Google had considered user experience in the design.
My first project was to create a “YouTube Title Writer” agent, a task that seemed straightforward enough to test the capabilities of Google Agent Builder.
The setup process involved defining the agent’s goal and instructions, which formed the core of its functionality.
Google helpfully provided some pre-filled text to guide me through this process, demonstrating an attempt to make the platform more accessible to newcomers.
Language Model Selection and Limitations
One of the most critical aspects of any AI agent is the underlying language model it uses.
Here, Google Agent Builder presented a disappointing reality.
The available language models were surprisingly outdated, lacking the cutting-edge options I had expected from a tech giant like Google.
Noticeably absent was Gemini 1.5 Pro, which had been announced alongside Google Agent Builder at the same conference.
This discrepancy between what was promised and what was delivered left me questioning the platform’s readiness for real-world applications.
After some deliberation, I settled on Gemini 1.0, the best available option at the time.
However, this choice felt like a compromise, especially considering the rapid advancements in AI language models.
The absence of more advanced models raised concerns about the agent’s potential performance and capabilities.
Crafting the Agent’s Behavior
Defining Goals and Instructions
With the foundation laid, I focused on fine-tuning my YouTube Title Writer agent.
Google Agent Builder allowed me to set specific goals and instructions, which would guide the agent’s behavior.
I defined the primary goal as creating “interesting and clickable titles,” a straightforward objective for a title-generating AI.
The instructions section offered more flexibility, allowing me to outline the agent’s workflow and response patterns.
I specified that the agent should greet the user, ask how it can help, and then summarize the user’s request before proceeding with title generation.
This level of customization was encouraging, suggesting that Google Agent Builder could potentially cater to a wide range of use cases.
Exploring Multi-Agent Possibilities
An intriguing feature of Google Agent Builder was the apparent ability to create multi-agent teams.
This concept piqued my interest, as it hinted at the potential for more complex AI systems.
I envisioned creating a secondary agent to analyze video ideas, complementing the title writer’s functions.
However, as I delved deeper into this possibility, I encountered limitations.
The platform didn’t seem fully prepared for seamless multi-agent interactions, requiring workarounds and additional setup steps.
This experience highlighted both the promise and the current constraints of Google Agent Builder.
Testing and Refining the Agent
The Importance of Examples
One crucial aspect of agent development in Google Agent Builder is the use of examples.
These serve as effective few-shot prompts, guiding the agent’s behavior and responses.
I created several examples, including one for “reading AI books,” to help shape the agent’s understanding of good title generation.
The process of creating these examples was somewhat unintuitive, with confusing terminology like “utterance” instead of more user-friendly terms.
This choice of language seemed to reflect a disconnect between the platform’s developers and its potential users.
Confronting Performance Issues
With examples in place, I eagerly began testing my YouTube Title Writer agent.
The results were, to put it mildly, underwhelming.
Despite clear instructions and examples, the agent often struggled to produce relevant or creative titles.
Its responses frequently ignored the provided context or generated titles that bore little resembance to the quality I had demonstrated in the examples.
This poor performance was particularly surprising given Google’s reputation in AI and natural language processing.
To better understand the issue, I experimented with different language models, including the less capable Gemma 2 billion parameter model.
Shockingly, the results were not significantly different, raising serious questions about the effectiveness of Google Agent Builder’s integration with its language models.
Attempting Multi-Agent Collaboration
Creating a Secondary Agent
Undeterred by the initial setbacks, I decided to explore the multi-agent capabilities of Google Agent Builder.
I created a second agent, an “Idea Analyst,” designed to objectively analyze video ideas provided by users.
The process of creating this agent mirrored that of the title writer, allowing me to define its goals and instructions separately.
This modular approach to agent creation showed promise, hinting at the potential for building more complex AI systems within the platform.
Integration Challenges
Integrating the Idea Analyst with the YouTube Title Writer proved to be a challenging endeavor.
The syntax for calling one agent from another was not immediately clear, requiring several attempts and adjustments.
Even after seemingly correct setup, the agents often failed to communicate effectively.
The title writer would generate titles without consulting the analyst, or the analyst would claim inability to critique ideas without context, despite clear instructions to the contrary.
These integration issues highlighted significant limitations in Google Agent Builder’s current capabilities for creating sophisticated, multi-agent systems.
Reflections on Google Agent Builder
Enterprise Readiness in Question
Throughout my experience with Google Agent Builder, one question repeatedly surfaced: Is this platform truly “Enterprise ready” as claimed?
The numerous challenges I encountered, from basic usability issues to performance limitations, cast doubt on this assertion.
For large companies with thousands of employees, the current state of Google Agent Builder seems far from ideal.
The platform’s inability to consistently produce useful results or facilitate smooth multi-agent interactions would likely frustrate rather than empower enterprise users.
Comparing to Alternatives
In light of these experiences, it’s natural to consider how Google Agent Builder stacks up against other AI agent development platforms.
Tools like Crew AI, LangGraph, and AutoGen offer more robust and flexible options for building functional AI agents.
These alternatives often provide better performance, more intuitive interfaces, and greater capabilities for complex agent interactions.
For those serious about AI agent development, exploring these other platforms might prove more fruitful than struggling with the current limitations of Google Agent Builder.
Conclusion
My journey with Google Agent Builder was an eye-opening experience, revealing both the potential and the current shortcomings of this much-hyped platform.
While Google’s ambition in the AI agent space is commendable, the execution leaves much to be desired.
From accessibility issues and outdated language models to performance inconsistencies and integration challenges, Google Agent Builder falls short of its promise as an enterprise-ready solution.
For now, those looking to develop effective AI agents might find more success with alternative platforms that offer greater functionality and ease of use.
As the field of AI continues to evolve rapidly, it’s clear that Google has work to do if it wants Google Agent Builder to compete with more established and capable tools in the market.
The potential is there, but realizing it will require significant improvements and a closer alignment with user needs and expectations.
Frequently Asked Questions (FAQ)
What is Google AI agent builder?
Google AI agent builder is a platform designed by Google to create and deploy AI-powered conversational agents.
It allows developers and businesses to build custom AI agents that can interact with users, perform tasks, and provide information.
The tool is part of Google’s suite of AI services, aimed at simplifying the process of creating intelligent, conversational interfaces.
Google AI agent builder utilizes Google’s language models and machine learning capabilities to enable natural language understanding and generation.
It’s intended to be used for various applications, including customer service, information retrieval, and task automation.
However, as my experience showed, its current implementation may have limitations in terms of performance and usability.
How do I create a Google agent?
Creating a Google agent involves several steps using the Google Agent Builder platform:
- Access Google Cloud: Log into your Google Cloud account.
- Navigate to Agent Builder: Find the Agent Builder option under “Apps” and then “Chat, recommendations, and agent”.
- Set up a new agent: Click on “Create a new app” and select your preferred region (note that some regions may not be available).
- Define your agent’s purpose: Specify the goal and instructions for your agent.
- Choose a language model: Select from the available language models (e.g., Gemini 1.0).
- Create examples: Provide sample interactions to guide your agent’s behavior.
- Add tools and integrations: If needed, incorporate additional functionalities like code interpreters.
- Test and refine: Use the testing interface to interact with your agent and make necessary adjustments.
- Deploy: Once satisfied with your agent’s performance, deploy it for use.
Remember that the process may be subject to changes as Google updates the platform.
How much does Google AI agent cost?
The cost of using Google AI agent builder can vary depending on several factors:
- Usage: Pricing is often based on the number of API calls or interactions your agent handles.
- Chosen language model: More advanced models may come with higher costs.
- Additional features: Integrating extra tools or services might incur additional charges.
- Enterprise agreements: Large-scale or enterprise users may have custom pricing structures.
As of my last update, Google had not publicly released a standardized pricing structure for Google AI agent builder.
It’s best to check Google’s official pricing page or contact their sales team for the most up-to-date and accurate pricing information.
Keep in mind that Google often offers free tiers or trial periods for their cloud services, which might apply to the AI agent builder as well.
What is Google generative AI App Builder?
Google Generative AI App Builder is a broader platform that encompasses various AI-powered tools for creating applications.
It’s designed to help developers and businesses build AI-enhanced applications quickly and efficiently.
The platform leverages Google’s advanced AI and machine learning capabilities, including natural language processing and generation.
Key features of Google Generative AI App Builder often include:
- Conversational AI tools, including the Google AI agent builder
- Text and code generation capabilities
- Integration with other Google Cloud services
- Tools for customizing and fine-tuning AI models
- Options for deploying AI-powered applications at scale
While it shares some similarities with Google AI agent builder, the App Builder is a more comprehensive suite of tools for various AI application development needs.
It’s important to note that Google frequently updates and rebrands its AI offerings, so the exact features and naming conventions may evolve over time.
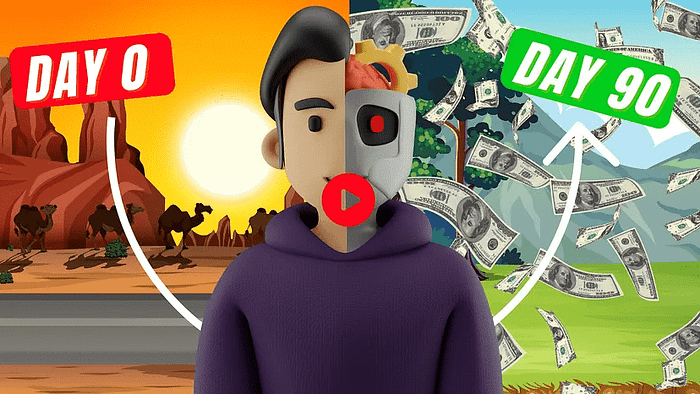
We strongly recommend that you check out our guide on how to take advantage of AI in today’s passive income economy.