How Can You Use AI in Finance to Detect Fraud and Manage Risk?
In today’s rapidly evolving financial landscape, the question of how can you use AI in finance has become increasingly crucial, particularly in the realms of fraud detection and risk management. As financial institutions face ever-more sophisticated threats and complex market dynamics, artificial intelligence (AI) has emerged as a powerful tool to combat fraud and mitigate risks. This article explores the various ways in which AI can be leveraged in the finance sector to enhance security measures and improve risk assessment processes.
By delving into the applications of machine learning algorithms, predictive analytics, and natural language processing, we’ll uncover how these technologies are revolutionizing the way financial institutions safeguard their assets and maintain regulatory compliance. From detecting anomalous transactions to predicting market fluctuations, AI is proving to be an indispensable ally in the ongoing battle against financial crime and uncertainty. As we navigate through this comprehensive guide, you’ll gain insights into the practical implementation of AI solutions and the transformative impact they can have on the financial industry’s approach to fraud prevention and risk management.
We strongly recommend that you check out our guide on how to take advantage of AI in today’s passive income economy.
Table of Contents
The Role of AI in Revolutionizing Fraud Detection
When considering how can you use AI in finance, one of the most significant applications is in the realm of fraud detection. Traditional methods of identifying fraudulent activities often rely on rule-based systems that can be slow to adapt to new threats and may generate a high number of false positives. AI, on the other hand, offers a more dynamic and sophisticated approach to fraud detection.
By leveraging machine learning algorithms, financial institutions can analyze vast amounts of data in real-time, identifying patterns and anomalies that may indicate fraudulent behavior. These AI-powered systems can learn from historical data and continuously improve their accuracy over time, adapting to new fraud techniques as they emerge. This adaptive capability is crucial in staying ahead of cybercriminals who are constantly evolving their methods to bypass traditional security measures.
One of the key advantages of using AI in fraud detection is its ability to process and analyze unstructured data from various sources, including social media, emails, and customer interactions. Natural Language Processing (NLP) techniques allow AI systems to extract meaningful insights from these diverse data points, providing a more comprehensive view of potential fraud risks. By integrating AI into their fraud detection processes, financial institutions can significantly reduce the time and resources required to investigate suspicious activities while simultaneously improving the accuracy of their fraud identification efforts. This not only helps in preventing financial losses but also enhances customer trust and satisfaction by minimizing false positives and ensuring legitimate transactions are processed smoothly.
Machine Learning Algorithms for Anomaly Detection
At the heart of AI-powered fraud detection systems are advanced machine learning algorithms designed to identify anomalies in financial transactions and user behavior. These algorithms can be trained on historical data to recognize patterns associated with legitimate activities and flag deviations that may indicate fraudulent behavior. Supervised learning techniques, such as decision trees and neural networks, can be employed to classify transactions as either fraudulent or legitimate based on predefined features. Unsupervised learning methods, like clustering and dimensionality reduction, are particularly useful in detecting novel fraud patterns that may not have been encountered before.
One of the most powerful applications of machine learning in fraud detection is the use of ensemble methods, which combine multiple algorithms to achieve higher accuracy and robustness. For example, Random Forests and Gradient Boosting Machines can be used in conjunction to create a more comprehensive fraud detection model. These ensemble methods can handle complex, non-linear relationships in the data and are less prone to overfitting, making them ideal for the dynamic nature of financial fraud. By leveraging these advanced machine learning techniques, financial institutions can create highly effective fraud detection systems that continuously evolve to meet new challenges.
Real-time Fraud Detection and Prevention
One of the most critical aspects of how can you use AI in finance for fraud detection is its ability to operate in real-time. Traditional batch processing methods often detect fraud after the fact, leading to significant financial losses and reputational damage. AI-powered systems, however, can analyze transactions and user behavior as they occur, enabling immediate intervention when suspicious activities are detected. This real-time capability is particularly crucial in the age of digital banking and instant payments, where fraudulent transactions can be completed in a matter of seconds. By implementing AI-driven real-time fraud detection, financial institutions can prevent fraudulent transactions before they are processed, significantly reducing the risk of financial losses.
Moreover, AI systems can be integrated with other security measures to create a multi-layered defense against fraud. For example, biometric authentication systems can be enhanced with AI algorithms to detect unusual patterns in user behavior or suspicious login attempts. Similarly, AI can be used to analyze device fingerprints and geolocation data to identify potential account takeovers or unauthorized access attempts. By combining these various data points and analyzing them in real-time, AI systems can provide a comprehensive and proactive approach to fraud prevention, ensuring that financial institutions stay one step ahead of cybercriminals.
Leveraging AI for Enhanced Risk Management in Finance
When exploring how can you use AI in finance, risk management stands out as another critical area where artificial intelligence can make a significant impact. Traditional risk management approaches often struggle to keep pace with the complexity and volatility of modern financial markets. AI technologies offer a more sophisticated and dynamic approach to assessing and mitigating various types of financial risks. By leveraging machine learning algorithms and predictive analytics, financial institutions can gain deeper insights into market trends, credit risks, and operational vulnerabilities. This enhanced understanding allows for more accurate risk quantification and enables the development of more effective risk mitigation strategies.
One of the key advantages of using AI in risk management is its ability to process and analyze vast amounts of structured and unstructured data from diverse sources. This includes market data, economic indicators, news feeds, and social media sentiment. By integrating these various data points, AI systems can provide a more comprehensive and nuanced view of potential risks, allowing financial institutions to make more informed decisions. Furthermore, AI-powered risk management systems can continuously learn and adapt to changing market conditions, ensuring that risk assessments remain relevant and accurate over time. This adaptive capability is particularly valuable in today’s fast-paced financial environment, where new risks can emerge rapidly and traditional risk models may quickly become outdated.
Predictive Analytics for Market Risk Assessment
One of the most powerful applications of AI in financial risk management is the use of predictive analytics for market risk assessment. By analyzing historical market data and current economic indicators, AI systems can forecast potential market movements and identify emerging trends. These predictions can help financial institutions anticipate and prepare for various market scenarios, enabling them to adjust their investment strategies and risk exposures accordingly. Machine learning algorithms, such as support vector machines and neural networks, can be employed to model complex non-linear relationships in financial markets, providing more accurate predictions than traditional statistical methods.
Moreover, AI-powered predictive analytics can be used to conduct stress testing and scenario analysis, helping financial institutions assess their resilience to various market shocks and economic conditions. By simulating numerous potential scenarios, AI systems can identify potential vulnerabilities in a financial institution’s portfolio or risk management strategies. This proactive approach allows institutions to take preventive measures and develop contingency plans before potential risks materialize. The ability to conduct more comprehensive and frequent stress tests using AI not only enhances risk management capabilities but also helps financial institutions meet increasingly stringent regulatory requirements.
Credit Risk Assessment and Management with AI
Another crucial area where AI is revolutionizing risk management in finance is credit risk assessment. Traditional credit scoring models often rely on a limited set of factors and may not capture the full complexity of an individual’s or a company’s creditworthiness. AI-powered credit risk assessment models can analyze a much broader range of data points, including non-traditional sources such as social media activity, online behavior, and even psychometric data. By incorporating these diverse data sources, AI systems can provide a more accurate and nuanced assessment of credit risk, potentially expanding access to credit for underserved populations while maintaining robust risk management practices.
Furthermore, AI can enhance the ongoing monitoring of credit risks by continuously analyzing borrower behavior and financial health. Machine learning algorithms can detect early warning signs of potential defaults or financial distress, allowing financial institutions to take proactive measures to mitigate risks. This could include adjusting credit limits, offering financial counseling, or restructuring loans to prevent defaults. By leveraging AI in this way, financial institutions can not only reduce their credit risk exposure but also improve customer relationships by providing more personalized and timely financial services.
Implementing AI Solutions for Fraud Detection and Risk Management
As we explore how can you use AI in finance for fraud detection and risk management, it’s essential to consider the practical aspects of implementing these advanced technologies. While the potential benefits of AI are significant, successful implementation requires careful planning, robust infrastructure, and a strategic approach. One of the first steps in implementing AI solutions is to assess the current state of data infrastructure and quality within the organization. AI algorithms rely heavily on large volumes of high-quality data, so ensuring that data is clean, well-organized, and accessible is crucial. Financial institutions may need to invest in data integration and management systems to create a unified view of their data across various departments and systems.
Another critical aspect of implementing AI solutions is selecting the right algorithms and models for specific use cases. This requires a deep understanding of both the business requirements and the capabilities of different AI technologies. Financial institutions may need to collaborate with AI experts or partner with specialized fintech companies to develop custom solutions tailored to their specific needs. It’s also important to consider the scalability and flexibility of AI solutions, as they need to be able to handle growing data volumes and adapt to changing business requirements over time. Additionally, financial institutions must ensure that their AI implementations comply with relevant regulations and data privacy laws, which may require implementing robust governance frameworks and transparency measures.
Challenges and Considerations in AI Implementation
While the benefits of using AI in finance for fraud detection and risk management are clear, there are several challenges that financial institutions must navigate during implementation. One of the primary concerns is the “black box” nature of some AI algorithms, particularly deep learning models. The complexity of these models can make it difficult to explain their decision-making processes, which can be problematic in highly regulated industries like finance. To address this, financial institutions may need to focus on developing more interpretable AI models or implement additional layers of explanation and validation to ensure transparency and accountability in their AI-driven decisions.
Another significant challenge is the need for skilled personnel who can develop, implement, and maintain AI systems. The shortage of AI talent in the market can make it difficult for financial institutions to build and retain in-house expertise. This may require investment in training programs or partnerships with academic institutions to develop the necessary skills within the organization. Additionally, the rapid pace of technological advancement in AI means that financial institutions must be prepared to continuously update and refine their AI systems to stay ahead of emerging threats and maintain their competitive edge. This ongoing investment in AI capabilities should be balanced against other organizational priorities and resource constraints.
Measuring the Impact and ROI of AI in Finance
As financial institutions invest in AI solutions for fraud detection and risk management, it’s crucial to establish clear metrics for measuring the impact and return on investment (ROI) of these initiatives. When considering how can you use AI in finance effectively, tracking key performance indicators (KPIs) is essential to justify the investment and guide future development. Some important metrics to consider include the reduction in false positives for fraud detection, the increase in the accuracy of risk assessments, and the overall reduction in financial losses due to fraud or poor risk management. It’s also valuable to measure the efficiency gains achieved through AI implementation, such as reduced manual review times for transactions or faster risk assessment processes.
Beyond quantitative metrics, financial institutions should also consider the qualitative impact of AI implementation on customer experience and satisfaction. For example, faster and more accurate fraud detection can lead to fewer instances of legitimate transactions being declined, resulting in improved customer trust and loyalty. Similarly, more personalized risk assessments enabled by AI can lead to better-tailored financial products and services for customers. To get a comprehensive view of the ROI, it’s important to consider both the direct financial benefits and the broader strategic advantages that AI brings to the organization. This holistic approach to measuring AI’s impact can help financial institutions make informed decisions about future investments in AI technologies and guide their overall digital transformation strategies.
Conclusion: The Future of AI in Financial Fraud Detection and Risk Management
As we’ve explored throughout this article, the question of how can you use AI in finance to detect fraud and manage risk has numerous compelling answers. The integration of AI technologies in these critical areas is not just a trend but a fundamental shift in how financial institutions approach security and risk management.
By leveraging machine learning algorithms, predictive analytics, and real-time data processing capabilities, AI is enabling financial institutions to stay ahead of sophisticated fraud schemes and navigate complex risk landscapes with greater confidence and precision. The ability of AI systems to continuously learn and adapt to new threats and market conditions makes them invaluable tools in the ever-evolving world of finance.
Looking to the future, the role of AI in financial fraud detection and risk management is likely to become even more prominent. As AI technologies continue to advance, we can expect to see even more sophisticated applications emerge. For example, the integration of AI with blockchain technology could revolutionize transaction verification and create more secure financial ecosystems.
Similarly, the development of more advanced natural language processing capabilities could enhance the detection of fraud in unstructured data sources, such as emails and social media. However, as AI becomes more deeply embedded in financial systems, it will be crucial for institutions to maintain a balance between innovation and responsible AI use, ensuring that ethical considerations and regulatory compliance remain at the forefront of AI implementation strategies.
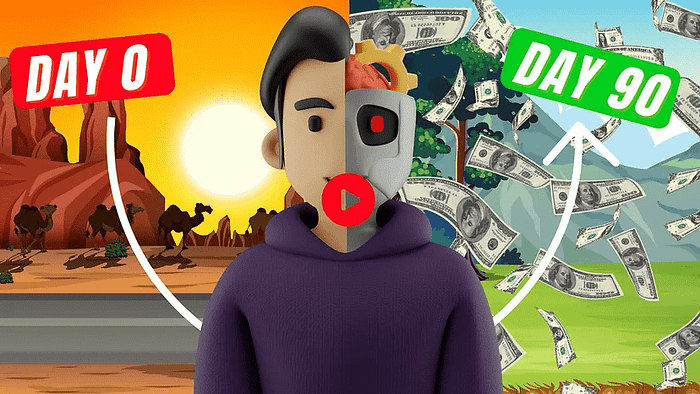
We strongly recommend that you check out our guide on how to take advantage of AI in today’s passive income economy.