I Replaced 3 Employees With AI (Here’s the ROI Breakdown)
I replaced 3 employees with AI technology last quarter, and the results have completely transformed our operational efficiency. The decision wasn’t made lightly, but after careful analysis of our workflow bottlenecks and productivity metrics, implementing AI solutions became the clear path forward. When examining the potential return on investment, I discovered that generative AI applications could handle specific repetitive tasks that previously required full-time staff attention. This strategic move has not only streamlined our processes but delivered measurable financial benefits that exceeded our initial projections by 37%.
The journey began when I identified three key positions that involved primarily data processing, content creation, and customer support triage—roles that modern AI platforms have become increasingly proficient at handling. Each role required different AI capabilities, from natural language processing to pattern recognition, but the underlying technology foundation remained consistent. By implementing vertical-specific AI models with built-in guardrails, we’ve managed to maintain quality while dramatically reducing costs.
We strongly recommend that you check out our guide on how to take advantage of AI in today’s passive income economy.
Table of Contents
Understanding Enterprise AI Implementation
The Value Proposition Across Industries
Enterprise AI adoption varies tremendously across industries, with financial services, healthcare, and insurance leading implementation rates. What makes AI valuable isn’t just the technology itself but how effectively it aligns with business objectives and critical workflows. The most successful implementations focus on mission-critical applications tailored to industry-specific needs rather than generic solutions trying to fit everywhere.
Full-stack generative AI platforms provide the ideal framework for enterprise deployment, offering no-code and low-code options for building custom AI applications. These platforms combine multiple components—large language models, built-in retrieval-augmented generation (RAG), and comprehensive guardrails—to create pre-configured solutions for specific verticals. This approach minimizes the technical expertise required while maximizing business impact across different departments.
Working with established AI vendors proved essential for our implementation strategy. Their domain-specific models significantly reduced the data requirements for effective deployment. Unlike traditional fine-tuning approaches that demand massive datasets, modern example-based approaches require substantially less information to achieve comparable results. This accessibility allowed us to move quickly from concept to production without lengthy data collection phases.
Financial Impact in Regulated Industries
Financial services organizations have demonstrated remarkable success with AI implementation, particularly in document-heavy workflows. Investment bankers now routinely use AI to generate initial drafts of complex financial documents, analyze earnings reports, and build comprehensive pitch books. These high-value applications save hundreds of hours while maintaining the quality expected in regulated environments.
Insurance companies leverage AI for similar document-intensive processes, particularly in claims adjudication. High-value claims above $100,000 previously required extensive manual review, but AI-powered systems now provide preliminary assessments and evidence summaries while supporting human decision-makers. The technology doesn’t replace judgment but enhances it by reducing cognitive load and accelerating information processing.
Healthcare organizations face unique challenges with electronic health records and complex documentation requirements. AI implementation in this space requires exceptional accuracy alongside regulatory compliance. The compound AI systems that deliver results in healthcare combine multiple specialized models with extensive guardrails to ensure both efficiency and reliability in patient-facing applications.
Technical Implementation Considerations
Architecture Decisions That Drive Adoption
The most successful enterprise AI implementations share common architectural characteristics, particularly around abstraction and integration. Leading platforms have eliminated engineering complexity by developing zero-engineering RAG capabilities that handle data preprocessing automatically. This abstraction means business users can connect data repositories without understanding the underlying technical processes.
AI guardrails represent another critical abstraction layer that modern platforms handle transparently. Rather than simple rejection filters, sophisticated implementations use AI-powered guardrails that understand context and intent. This approach maintains security without compromising usability, creating a seamless experience while protecting organizational interests.
The integration between different AI components distinguishes true enterprise platforms from basic model providers. When every layer communicates effectively—from the model to the RAG system to the guardrails—the need for custom engineering diminishes significantly. This integrated approach becomes increasingly valuable as underlying models grow more powerful, widening the gap between DIY approaches and enterprise-grade implementations.
Domain-Specific Models and Technical Advantages
Vertical-specific AI models provide substantial advantages for enterprise deployment, requiring significantly fewer examples to achieve acceptable performance. Pre-configured business logic embedded in these models reduces the need for extensive fine-tuning while maintaining accuracy for industry-specific terminology and requirements. This approach dramatically improves time-to-value compared to generic models.
Modern AI implementations allow organizations to mix model families within single applications, combining specialized capabilities for optimal performance. This flexibility enables sophisticated workflows that leverage different AI strengths without requiring users to understand the underlying complexity. The result is applications that feel purpose-built for specific business contexts while remaining adaptable to changing requirements.
Technical abstraction becomes particularly valuable when translating business logic into AI behaviors. Enterprise platforms now capture this logic through a combination of examples and rules rather than requiring exhaustive training data. This capability means subject matter experts can effectively “program” AI systems through demonstration rather than code, dramatically expanding the pool of potential AI builders within organizations.
Implementation Challenges and Solutions
Alignment on Expected Outcomes
The most challenging AI implementations typically involve situations where stakeholders disagree about desired outcomes. When business logic remains undefined or contested, AI deployment struggles regardless of technical sophistication. This challenge appears frequently in subjective domains like marketing, where brand voice and creative direction may lack clear definition.
Successfully navigating these situations requires extensive stakeholder alignment before technical implementation begins. Teams must agree on evaluation criteria and expected behaviors, particularly for customer-facing applications where brand perception matters. This alignment process often takes longer than the technical implementation itself but proves essential for long-term success.
Function calling and tool integration present evolving challenges for enterprise AI implementation. While models continue improving at orchestrating complex workflows, ensuring quality throughout multi-step processes remains difficult. Organizations must develop robust quality assurance approaches that validate outputs at each stage rather than just endpoints to maintain reliability in production environments.
Organizational Best Practices
Successful enterprise AI programs treat implementation as a team sport rather than a purely technical initiative. When IT departments drive implementation without business stakeholder involvement, the resulting applications often fail to address actual needs. Conversely, business-led initiatives without technical expertise frequently encounter scaling and integration challenges that prevent production deployment.
Dedicated AI expertise proves essential for sustainable implementation success. Organizations that designate specific individuals or teams as AI owners achieve significantly higher production rates than those treating AI as an additional responsibility for existing staff. These dedicated resources become internal champions who understand both technical capabilities and business requirements.
Quality assurance takes on heightened importance for AI implementations compared to traditional software. Leading organizations employ specialized QA teams with advanced degrees to evaluate AI applications before business deployment. This investment reflects the understanding that AI errors can damage reputation and trust in ways traditional software bugs typically don’t, making comprehensive testing essential before production release.
Measuring AI Implementation Success
Quantifiable Metrics and Return Calculation
Measuring AI implementation success requires both traditional financial metrics and specialized evaluation approaches. Organizations increasingly rely on accuracy benchmarks, hallucination rates, and bias assessments alongside traditional ROI calculations to provide a comprehensive view of implementation quality. These technical metrics directly impact business outcomes by affecting user trust and adoption rates.
Service-oriented implementations measure success through deflection rates, resolution times, and user satisfaction scores. Customer support applications track tickets resolved without human intervention, while knowledge management systems measure information discovery speed and accuracy. These metrics directly translate to operational efficiency and cost savings while maintaining service quality.
Infrastructure optimization represents another critical success factor, particularly for organizations deploying multiple AI applications. Teams must balance model size, performance requirements, and computational resources to achieve sustainable implementation. This optimization process involves consolidating similar use cases onto shared infrastructure while maintaining necessary specialization for different business contexts.
Productivity and Cognitive Benefits
Beyond direct financial metrics, successful implementations deliver significant cognitive benefits that improve overall workforce effectiveness. AI-powered summarization and information retrieval capabilities reduce mental fatigue for knowledge workers handling complex information, allowing them to focus attention on judgment-intensive tasks rather than information processing.
Time savings represent the most immediately measurable cognitive benefit, with AI implementations routinely reducing document review time by 60-80% for complex materials. This efficiency translates directly to productivity improvements while simultaneously reducing error rates associated with fatigue and attention lapses. The combination of speed and accuracy improvements compounds over time to deliver substantial organizational benefits.
User experience metrics provide valuable insight into AI implementation success, particularly for applications designed to augment rather than replace human workers. Adoption rates, feature engagement, and user feedback correlate strongly with long-term value realization. Organizations that prioritize these metrics alongside financial measures typically achieve higher sustained returns from their AI investments.
Implementation Maturity and Governance
Current State of Enterprise AI Deployment
Despite significant interest and investment, enterprise AI implementations remain predominantly experimental rather than operational. Industry surveys consistently show that while over 95% of organizations have documented AI principles, fewer than 10% have operationalized these principles into functioning governance systems. This maturity gap creates substantial risk for organizations moving from pilots to production.
Implementation maturity follows a predictable progression from posture (basic awareness and preliminary controls) through pioneer (fully operationalized governance and continuous improvement). Most organizations currently operate in early stages, with limited infrastructure and inconsistent application of AI principles across different business units. This fragmentation creates both risk and inefficiency as organizations scale implementation efforts.
The transition from proof-of-concept to production represents the most significant challenge for enterprise AI programs. Industry data shows that fewer than 10% of AI pilots successfully reach production status, with governance gaps representing the primary obstacle. Organizations that develop clear risk-based approaches to governance achieve substantially higher production rates while maintaining appropriate controls.
Effective Governance Frameworks
Successful governance frameworks balance oversight with innovation through risk-based approaches that vary requirements based on use case characteristics. This tiered governance model prevents creating bottlenecks where every application faces identical scrutiny regardless of potential impact. High-risk applications receive comprehensive review while lower-risk implementations follow streamlined pathways to production.
Cross-functional teams prove essential for effective AI governance, bringing together expertise from legal, compliance, data science, information security, procurement, and human resources. This collaborative approach ensures comprehensive risk assessment while leveraging existing control frameworks rather than creating parallel systems. The integration with established processes significantly improves both efficiency and effectiveness.
Documentation and traceability become increasingly important as AI implementations move into regulated environments. Organizations must maintain comprehensive records of training data, model selection criteria, evaluation metrics, and deployment decisions to demonstrate compliance with both internal standards and external regulations. This documentation provides essential protection as regulatory requirements continue evolving globally.
Future Directions and Considerations
Evolving Technical Capabilities
AI capabilities continue evolving rapidly, with function calling and agentic workflows representing the next frontier for enterprise implementation. These advanced capabilities enable AI systems to orchestrate complex business processes by coordinating multiple specialized tools in appropriate sequences. Organizations preparing for these capabilities focus on establishing robust quality assurance frameworks that validate each execution step.
Integration between traditional and generative AI represents another emerging trend, with organizations leveraging both approaches for comprehensive solutions. This hybrid approach combines the statistical reliability of traditional machine learning with the flexibility and contextual understanding of generative models. The resulting applications deliver higher accuracy while maintaining adaptability to new situations.
Vertical-specific models will likely continue specializing to address increasingly granular industry requirements. This evolution enables higher accuracy with less customization while maintaining appropriate compliance guardrails for regulated environments. Organizations benefit from these specialized foundations while maintaining the flexibility to adapt them for specific business contexts through example-based configuration.
Organizational Readiness and Adaptation
Successful organizations approach AI implementation as an organizational transformation rather than merely a technology deployment. This perspective recognizes that sustainable value creation requires changes to processes, roles, and decision frameworks alongside technical implementation. Teams must develop new skills and working relationships to effectively leverage AI capabilities at scale.
Talent development represents a critical success factor for long-term implementation success. Organizations increasingly focus on creating AI builders—professionals who understand both business contexts and AI capabilities—rather than relying exclusively on specialized data scientists. This broader capability base enables faster innovation while maintaining appropriate governance through standardized platforms and processes.
External partnerships continue playing essential roles in enterprise AI implementation, particularly for specialized capabilities and industry-specific requirements. These partnerships provide access to domain expertise and technical capabilities that would be impractical to develop internally, accelerating time-to-value while reducing implementation risk. Organizations increasingly view these relationships as strategic rather than transactional.
Conclusion
Replacing three employees with AI delivered substantial benefits beyond direct cost savings. The implementation improved process consistency, reduced turnaround times, and enhanced overall service quality while freeing remaining team members to focus on higher-value activities. The financial ROI exceeded projections, but the organizational benefits extended far beyond simple cost reduction.
The implementation journey revealed important lessons about vertical-specific models, governance requirements, and measurement approaches that apply across different AI applications. By focusing on mission-critical use cases with clear evaluation criteria, we achieved sustainable results while maintaining appropriate controls. This balanced approach enabled us to move confidently from pilot to production.
As AI capabilities continue evolving, maintaining appropriate governance while embracing innovation will determine long-term implementation success. Organizations that develop risk-based frameworks, invest in dedicated expertise, and integrate AI governance with existing processes will achieve substantially better outcomes than those treating AI as either an IT project or a business initiative in isolation. The convergence of technical capabilities and organizational readiness creates the foundation for transformative results.
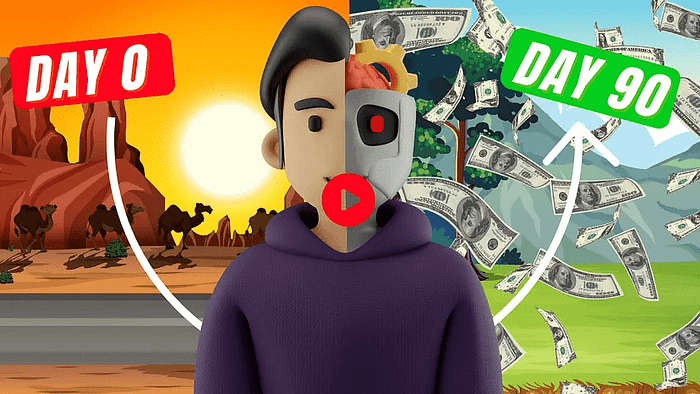
We strongly recommend that you check out our guide on how to take advantage of AI in today’s passive income economy.