How to Use AI in Financial Analysis to Predict Market Trends
In today’s rapidly evolving financial landscape, knowing how to use AI in financial analysis has become crucial for predicting market trends and gaining a competitive edge.
Artificial Intelligence (AI) has revolutionized the way we approach financial analysis, offering unprecedented insights and capabilities.
This article will explore the intricacies of leveraging AI in financial analysis to forecast market movements and make informed decisions.
We’ll delve into the various techniques, tools, and strategies that financial professionals can employ to harness the power of AI in their analytical processes.
By understanding how to use AI in financial analysis effectively, you’ll be better equipped to navigate the complexities of modern financial markets and stay ahead of the curve.
So, let’s embark on this journey to uncover the potential of AI in predicting market trends and transforming financial analysis as we know it.
We strongly recommend that you check out our guide on how to take advantage of AI in today’s passive income economy.
Table of Contents
The Fundamentals of AI in Financial Analysis
Before we dive into the specifics of using AI to predict market trends, it’s essential to grasp the basics of how AI is applied in financial analysis.
At its core, AI in financial analysis involves the use of advanced algorithms and machine learning techniques to process vast amounts of financial data and extract meaningful insights.
These AI-powered systems can analyze historical data, identify patterns, and make predictions with a level of speed and accuracy that far surpasses human capabilities.
By leveraging AI in financial analysis, analysts can automate many time-consuming tasks, reduce human error, and uncover hidden relationships within complex datasets.
This allows for more comprehensive and nuanced analysis, leading to better-informed decision-making and more accurate forecasts.
Understanding how to use AI in financial analysis begins with recognizing its potential to augment human expertise rather than replace it entirely.
Key Components of AI in Financial Analysis
To effectively use AI in financial analysis, it’s crucial to understand its key components and how they work together.
Machine Learning (ML) algorithms form the backbone of AI systems, enabling them to learn from data and improve their performance over time.
Natural Language Processing (NLP) allows AI to interpret and analyze textual data, such as news articles and social media posts, which can significantly impact market trends.
Deep Learning, a subset of ML, uses neural networks to model complex patterns and relationships within financial data.
Time Series Analysis is another critical component, particularly useful for predicting market trends based on historical data patterns.
By mastering these components, financial analysts can better understand how to use AI in financial analysis to its full potential.
Each of these elements plays a crucial role in the AI-driven analysis process, contributing to more accurate and insightful predictions.
Leveraging AI for Market Trend Prediction
Now that we’ve covered the basics, let’s explore how to use AI in financial analysis specifically for predicting market trends.
AI’s ability to process and analyze vast amounts of data in real-time makes it an invaluable tool for identifying emerging trends and potential market shifts.
By utilizing machine learning algorithms, AI systems can detect subtle patterns and correlations that human analysts might overlook, providing a more comprehensive view of market dynamics.
One of the key advantages of using AI in this context is its ability to consider a wide range of factors simultaneously, including economic indicators, geopolitical events, and even sentiment analysis from social media.
This holistic approach to data analysis allows for more nuanced and accurate predictions of market trends.
Moreover, AI systems can continuously learn and adapt to new information, refining their predictive models over time for improved accuracy.
Data Collection and Preprocessing
The first step in using AI for market trend prediction is gathering and preprocessing relevant data.
This involves collecting historical financial data, market indicators, economic reports, news articles, and other pertinent information.
It’s crucial to ensure that the data is clean, consistent, and properly formatted for analysis.
AI systems can assist in this process by automating data collection from various sources and identifying potential errors or inconsistencies.
Preprocessing may also involve normalizing data, handling missing values, and transforming variables to make them suitable for analysis.
By mastering this initial stage, analysts can lay a solid foundation for how to use AI in financial analysis effectively.
The quality and comprehensiveness of the input data directly impact the accuracy of the AI’s predictions, making this step critical to the overall process.
Feature Selection and Engineering
Once the data is prepared, the next step in learning how to use AI in financial analysis is feature selection and engineering.
This involves identifying the most relevant variables or “features” that are likely to influence market trends.
AI algorithms can assist in this process by analyzing the relationships between different variables and their impact on market movements.
Feature engineering may involve creating new variables based on existing data, such as moving averages or volatility indicators.
This step is crucial in enhancing the predictive power of AI models and improving their ability to forecast market trends accurately.
By carefully selecting and engineering features, analysts can ensure that their AI models focus on the most important factors driving market behavior.
This targeted approach leads to more reliable and actionable insights, demonstrating the value of knowing how to use AI in financial analysis.
AI Techniques for Market Trend Prediction
With the data prepared and features selected, it’s time to explore specific AI techniques for predicting market trends.
Understanding these techniques is essential for anyone looking to master how to use AI in financial analysis effectively.
One popular approach is the use of Recurrent Neural Networks (RNNs), particularly Long Short-Term Memory (LSTM) networks, which are well-suited for analyzing sequential data like time series.
These models can capture long-term dependencies in financial data, making them effective for predicting future market movements.
Another powerful technique is the use of ensemble methods, which combine multiple AI models to improve prediction accuracy and robustness.
By leveraging diverse AI techniques, analysts can develop more comprehensive and reliable predictive models for market trends.
Time Series Forecasting with ARIMA and Prophet
When learning how to use AI in financial analysis for trend prediction, it’s important to consider traditional time series forecasting methods enhanced by AI.
ARIMA (AutoRegressive Integrated Moving Average) models, when combined with machine learning techniques, can provide accurate short-term forecasts of market trends.
Facebook’s Prophet algorithm, designed for forecasting time series data, is another valuable tool in the AI-driven financial analysis toolkit.
These methods can be particularly effective for predicting seasonal patterns and cyclical trends in financial markets.
By incorporating these advanced time series techniques into their analysis, financial professionals can gain deeper insights into market behavior and trends.
The combination of traditional statistical methods and modern AI approaches often yields the most accurate and reliable predictions.
Sentiment Analysis for Market Trend Prediction
Another crucial aspect of using AI in financial analysis for market trend prediction is sentiment analysis.
AI-powered sentiment analysis tools can process vast amounts of textual data from news articles, social media, and financial reports to gauge market sentiment.
This information can be invaluable in predicting short-term market movements and potential trend reversals.
Natural Language Processing (NLP) techniques allow AI systems to understand the context and nuances of financial news and discussions.
By incorporating sentiment analysis into their predictive models, analysts can capture the psychological factors that often drive market behavior.
This holistic approach to market analysis demonstrates the power of knowing how to use AI in financial analysis comprehensively.
Implementing AI Models for Financial Analysis
With a solid understanding of AI techniques, the next step is implementing these models in your financial analysis workflow.
This process involves selecting the appropriate AI frameworks and tools, training your models on historical data, and integrating them into your existing analytical processes.
Popular frameworks for implementing AI in financial analysis include TensorFlow, PyTorch, and scikit-learn, each offering unique advantages for different types of analysis.
It’s crucial to choose the right tools based on your specific needs and the complexity of your predictive models.
Implementing AI models also requires careful consideration of computational resources, as some advanced models may require significant processing power.
By mastering the implementation process, you’ll be well-equipped to leverage AI in your financial analysis effectively.
Model Training and Validation
A critical step in learning how to use AI in financial analysis is properly training and validating your models.
This involves splitting your data into training, validation, and test sets to ensure that your models can generalize well to new, unseen data.
Cross-validation techniques can help assess the model’s performance and prevent overfitting, which is a common challenge in AI-driven financial analysis.
It’s important to regularly retrain and update your models with new data to maintain their accuracy and relevance.
Backtesting your AI models against historical data can provide valuable insights into their predictive power and potential limitations.
By mastering the art of model training and validation, you can ensure that your AI-driven financial analysis produces reliable and actionable insights.
Interpreting AI Model Outputs
Once your AI models are implemented and trained, the next crucial skill in using AI for financial analysis is interpreting their outputs.
AI models can generate complex predictions and insights, but translating these into actionable investment strategies requires careful interpretation.
It’s important to understand the limitations and potential biases of your AI models to avoid over-reliance on their predictions.
Visualization tools can be incredibly helpful in presenting AI model outputs in a clear and understandable format for decision-makers.
By combining AI-generated insights with human expertise and judgment, you can make more informed and balanced financial decisions.
Mastering the interpretation of AI model outputs is key to effectively using AI in financial analysis and leveraging its full potential.
Challenges and Considerations in Using AI for Financial Analysis
While AI offers tremendous potential for predicting market trends, it’s important to be aware of the challenges and considerations involved.
One of the primary challenges is the “black box” nature of some AI models, which can make it difficult to explain their decision-making process.
This lack of transparency can be problematic in regulated financial environments where decision rationales must be clearly explained.
Another consideration is the potential for AI models to perpetuate or amplify existing biases present in historical financial data.
It’s crucial to regularly monitor and audit AI systems to ensure they’re not making biased or unfair predictions.
Additionally, the rapidly changing nature of financial markets means that AI models must be continuously updated and refined to remain effective.
By understanding these challenges, you can approach AI in financial analysis with a balanced and informed perspective.
Ethical Considerations in AI-Driven Financial Analysis
As we explore how to use AI in financial analysis, it’s essential to consider the ethical implications of these powerful tools.
AI systems have the potential to significantly impact financial markets and individual investments, raising questions about fairness and accountability.
It’s important to ensure that AI-driven financial analysis doesn’t exacerbate existing inequalities or create new ones in the financial system.
Transparency in how AI models make predictions and recommendations is crucial for maintaining trust in financial institutions and markets.
There’s also the question of data privacy and security, as AI systems often require access to vast amounts of sensitive financial information.
By addressing these ethical considerations, we can ensure that AI is used responsibly and beneficially in financial analysis.
The Future of AI in Financial Analysis
As we look ahead, the role of AI in financial analysis is set to become even more prominent and sophisticated.
Advancements in areas such as quantum computing and edge AI are likely to open up new possibilities for real-time, hyper-accurate market predictions.
We can expect to see more integration of AI with other emerging technologies like blockchain and the Internet of Things (IoT) in financial analysis.
This convergence of technologies will likely lead to even more comprehensive and insightful market trend predictions.
The democratization of AI tools may also allow smaller investors and firms to leverage advanced financial analysis capabilities previously only available to large institutions.
As AI continues to evolve, those who master how to use AI in financial analysis will be well-positioned to navigate the complexities of future financial markets.
The key to success will be staying informed about the latest developments and continuously adapting your approach to AI-driven financial analysis.
Conclusion:
In conclusion, learning how to use AI in financial analysis to predict market trends is becoming an essential skill for financial professionals in the 21st century.
The power of AI to process vast amounts of data, identify complex patterns, and generate accurate predictions is transforming the landscape of financial analysis.
By mastering the fundamentals of AI, understanding various predictive techniques, and implementing robust AI models, analysts can gain a significant edge in forecasting market trends.
However, it’s crucial to approach AI-driven financial analysis with a balanced perspective, considering both its potential and limitations.
Ethical considerations and the need for transparent, explainable AI models should always be at the forefront of any AI implementation in finance.
As we look to the future, the integration of AI in financial analysis will only become more sophisticated and ubiquitous.
Those who invest in developing their skills in this area will be well-prepared to navigate the complex, data-driven financial markets of tomorrow.
By embracing AI as a powerful tool in their analytical arsenal, financial professionals can unlock new levels of insight and predictive power, ultimately leading to more informed decision-making and better financial outcomes.
Frequently Asked Questions
How is AI used in financial analysis?
AI is used in financial analysis in several ways:
- Predictive Analytics: AI algorithms analyze historical data to forecast future market trends and asset prices.
- Risk Assessment: AI models evaluate potential risks in investments and portfolios by processing vast amounts of data.
- Fraud Detection: AI systems can identify unusual patterns that may indicate fraudulent activities.
- Algorithmic Trading: AI-powered systems execute trades at optimal times based on market conditions.
- Natural Language Processing: AI analyzes news articles and social media to gauge market sentiment.
- Portfolio Management: AI assists in optimizing asset allocation and rebalancing portfolios.
- Customer Service: AI chatbots provide instant responses to customer queries about financial products and services.
- Credit Scoring: AI models assess creditworthiness more accurately by considering a wider range of factors.
How to use AI as a financial analyst?
To use AI as a financial analyst:
- Learn the Basics: Understand fundamental AI concepts and how they apply to finance.
- Master Data Analysis: Develop strong skills in data preprocessing and feature engineering.
- Choose the Right Tools: Familiarize yourself with AI platforms like Python, R, and TensorFlow.
- Understand AI Models: Learn about different AI algorithms and their applications in finance.
- Implement AI in Your Workflow: Start incorporating AI tools into your daily analysis tasks.
- Interpret Results: Develop the ability to interpret and explain AI-generated insights.
- Stay Updated: Keep abreast of the latest developments in AI and finance.
- Collaborate: Work with data scientists and AI experts to enhance your skills and knowledge.
Which AI tool is best for financial analysis?
The best AI tool for financial analysis depends on specific needs, but some popular options include:
- Python: Versatile programming language with extensive libraries for financial analysis and machine learning.
- R: Statistical programming language widely used in quantitative finance.
- TensorFlow: Open-source platform for machine learning, suitable for complex financial modeling.
- PyTorch: Another powerful open-source machine learning library.
- MATLAB: Numerical computing environment popular for financial modeling and algorithm development.
- Tableau: Data visualization tool that can integrate with AI models for financial reporting.
- H2O.ai: Provides a range of AI and machine learning tools for various financial applications.
- Bloomberg Terminal: Offers AI-powered analytics for market data and financial information.
How to use AI for financial modelling?
To use AI for financial modelling:
- Define Your Objective: Clearly outline what you want to achieve with your financial model.
- Collect and Prepare Data: Gather relevant financial data and preprocess it for AI analysis.
- Choose the Right AI Technique: Select appropriate AI algorithms based on your modelling needs (e.g., neural networks for complex non-linear relationships).
- Train Your Model: Use historical data to train your AI model.
- Validate and Test: Ensure your model performs well on new, unseen data.
- Interpret Results: Analyze the outputs of your AI model in the context of financial theory and market realities.
- Implement and Monitor: Integrate the AI model into your financial decision-making process and continuously monitor its performance.
- Refine and Update: Regularly update your model with new data and refine it based on performance feedback.
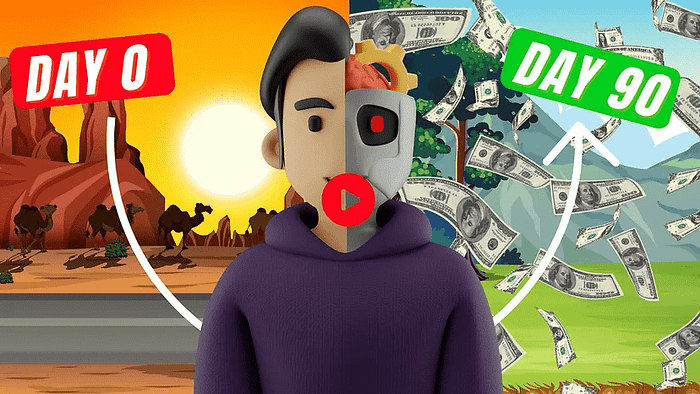
We strongly recommend that you check out our guide on how to take advantage of AI in today’s passive income economy.