How to Use AI in Financial Services: A Comprehensive Guide for Banks
In today’s rapidly evolving financial landscape, understanding how to use AI in financial services has become crucial for banks and financial institutions.
As technology continues to reshape the industry, artificial intelligence (AI) has emerged as a game-changing force, offering unprecedented opportunities for efficiency, innovation, and customer satisfaction.
This comprehensive guide will explore the various ways banks can harness the power of AI to transform their operations, enhance customer experiences, and stay competitive in an increasingly digital world.
From automating routine tasks to providing personalized financial advice, AI has the potential to revolutionize every aspect of banking.
By embracing AI technologies, financial institutions can streamline their processes, reduce costs, and deliver more value to their customers.
In this article, we’ll delve into the practical applications of AI in financial services, discussing best practices, potential challenges, and strategies for successful implementation.
Whether you’re a small community bank or a large multinational institution, this guide will provide valuable insights on how to leverage AI to drive growth and innovation in your organization.
We strongly recommend that you check out our guide on how to take advantage of AI in today’s passive income economy.
Table of Contents
The Foundations of AI in Financial Services
Understanding AI and Its Relevance to Banking
To effectively use AI in financial services, it’s essential to have a solid understanding of what AI is and how it applies to the banking sector.
At its core, AI refers to computer systems that can perform tasks that typically require human intelligence, such as visual perception, speech recognition, decision-making, and language translation.
In the context of financial services, AI encompasses a wide range of technologies, including machine learning, natural language processing, and computer vision.
These technologies can be applied to various aspects of banking, from customer service and fraud detection to risk assessment and investment management.
By leveraging AI, banks can analyze vast amounts of data, identify patterns, and make predictions with a level of accuracy and speed that surpasses human capabilities.
This ability to process and interpret complex information in real-time opens up new possibilities for how financial institutions can operate and serve their customers.
As we explore how to use AI in financial services, it’s important to recognize that AI is not a one-size-fits-all solution, but rather a versatile tool that can be tailored to meet specific needs and challenges within the banking industry.
Key AI Technologies Transforming Financial Services
When considering how to use AI in financial services, it’s crucial to familiarize yourself with the key technologies driving this transformation.
Machine learning, a subset of AI, is perhaps the most widely used technology in banking, enabling systems to learn from data and improve their performance over time without explicit programming.
Natural language processing (NLP) allows computers to understand, interpret, and generate human language, making it invaluable for chatbots, voice assistants, and document analysis.
Computer vision, which enables machines to interpret and analyze visual information, is increasingly being used for tasks such as identity verification and check processing.
Robotics process automation (RPA) combines AI with automation to handle repetitive tasks, freeing up human employees to focus on more complex and value-added activities.
Each of these technologies plays a crucial role in how banks can leverage AI to enhance their operations and services.
By understanding the capabilities and potential applications of these AI technologies, financial institutions can make informed decisions about how to integrate them into their existing systems and processes.
As we delve deeper into how to use AI in financial services, we’ll explore specific use cases for each of these technologies and discuss strategies for their successful implementation.
Practical Applications of AI in Banking
Customer Service and Engagement
One of the most visible ways banks are using AI in financial services is through enhanced customer service and engagement.
AI-powered chatbots and virtual assistants are revolutionizing how banks interact with their customers, providing 24/7 support and instant responses to inquiries.
These AI systems can handle a wide range of tasks, from answering frequently asked questions to guiding customers through complex transactions.
By leveraging natural language processing, these virtual assistants can understand and respond to customer queries in a natural, conversational manner.
Moreover, AI can analyze customer data to provide personalized recommendations and offers, enhancing the overall banking experience.
Machine learning algorithms can predict customer needs based on their behavior and financial history, allowing banks to proactively offer relevant products and services.
This level of personalization not only improves customer satisfaction but also increases the likelihood of cross-selling and upselling opportunities.
As banks continue to explore how to use AI in financial services, the focus on enhancing customer engagement through AI-driven solutions is likely to intensify.
Fraud Detection and Prevention
Another critical application of AI in financial services is in the area of fraud detection and prevention.
Traditional rule-based systems for detecting fraudulent activities are increasingly being replaced or augmented by more sophisticated AI-powered solutions.
Machine learning algorithms can analyze vast amounts of transaction data in real-time, identifying patterns and anomalies that may indicate fraudulent activity.
These AI systems can learn from new fraud patterns and adapt their detection methods accordingly, staying one step ahead of cybercriminals.
By using AI for fraud detection, banks can significantly reduce false positives, which can be a major source of frustration for customers and a drain on resources for financial institutions.
AI can also be used to enhance identity verification processes, using facial recognition and other biometric technologies to provide an additional layer of security.
As financial institutions continue to explore how to use AI in financial services, the development of more advanced fraud detection and prevention systems will remain a top priority.
Risk Assessment and Credit Scoring
AI is transforming how banks approach risk assessment and credit scoring, enabling more accurate and nuanced evaluations of creditworthiness.
Traditional credit scoring models often rely on a limited set of factors, but AI-powered systems can analyze a much broader range of data points to assess credit risk.
Machine learning algorithms can incorporate alternative data sources, such as social media activity and mobile phone usage, to build more comprehensive risk profiles.
This approach is particularly valuable for assessing the creditworthiness of individuals or businesses with limited credit histories, potentially expanding access to financial services.
AI can also help banks better predict and manage portfolio risk by analyzing market trends, economic indicators, and other relevant data sources.
By leveraging AI for risk assessment, banks can make more informed lending decisions, potentially reducing default rates and improving overall portfolio performance.
As financial institutions continue to explore how to use AI in financial services, the refinement of AI-driven risk assessment models is likely to be an ongoing area of focus and innovation.
Investment Management and Robo-Advisors
The use of AI in investment management has given rise to robo-advisors, automated platforms that provide algorithm-driven financial planning services with minimal human intervention.
These AI-powered advisors use machine learning algorithms to create and manage investment portfolios based on clients’ financial goals, risk tolerance, and market conditions.
Robo-advisors can continuously monitor and rebalance portfolios, making adjustments in response to market changes or shifts in the client’s financial situation.
This automated approach to investment management can provide cost-effective and accessible financial advice to a broader range of customers.
For traditional banks, incorporating robo-advisory services can be an effective way to expand their offerings and attract tech-savvy customers.
However, it’s important to note that while robo-advisors excel at certain tasks, they may not be suitable for all types of financial planning, particularly for high-net-worth individuals with complex financial needs.
As banks continue to explore how to use AI in financial services, finding the right balance between automated and human-led investment services will be crucial.
Regulatory Compliance and Anti-Money Laundering (AML)
Ensuring regulatory compliance and combating money laundering are critical challenges for financial institutions, and AI is proving to be a powerful tool in addressing these issues.
AI-powered systems can analyze vast amounts of data to identify potential compliance violations or suspicious activities that may indicate money laundering.
Machine learning algorithms can adapt to new regulatory requirements and emerging money laundering techniques, helping banks stay ahead of potential risks.
Natural language processing can be used to analyze unstructured data sources, such as emails and social media posts, to detect potential compliance issues or illicit activities.
By automating many aspects of compliance monitoring and reporting, AI can help banks reduce the risk of human error and allocate their compliance resources more efficiently.
This not only helps banks meet their regulatory obligations but also protects them from the reputational and financial risks associated with compliance failures.
As financial institutions continue to grapple with complex and evolving regulatory landscapes, the ability to effectively use AI in financial services for compliance purposes will become increasingly important.
Implementing AI in Financial Services: Strategies for Success
Developing an AI Strategy
To successfully use AI in financial services, banks need to develop a comprehensive AI strategy that aligns with their overall business objectives.
This strategy should identify specific areas where AI can add the most value, considering factors such as potential cost savings, revenue generation, and customer experience improvements.
It’s important to prioritize AI initiatives based on their potential impact and feasibility, taking into account the organization’s current capabilities and resources.
The AI strategy should also address key considerations such as data governance, ethical use of AI, and the need for ongoing monitoring and evaluation of AI systems.
Collaboration between IT, business units, and data science teams is crucial in developing an effective AI strategy that addresses both technical and business needs.
Banks should also consider partnering with fintech companies or technology providers to access specialized AI expertise and accelerate their AI initiatives.
By taking a strategic approach to how to use AI in financial services, banks can ensure that their AI investments deliver meaningful and sustainable value to the organization.
Building AI Capabilities and Infrastructure
Implementing AI in financial services requires banks to build the necessary capabilities and infrastructure to support AI-driven solutions.
This includes investing in robust data management systems to ensure that high-quality, relevant data is available to train and power AI models.
Banks need to develop or acquire the technical expertise required to design, implement, and maintain AI systems, which may involve hiring data scientists and AI specialists.
Cloud computing infrastructure is often essential for supporting the computational requirements of AI systems, offering scalability and flexibility.
It’s also important to establish governance frameworks and processes for managing AI projects, including protocols for testing, validating, and deploying AI models.
Banks should consider creating dedicated AI labs or centers of excellence to foster innovation and experimentation with AI technologies.
By building a strong foundation of AI capabilities and infrastructure, banks can position themselves to effectively leverage AI across their operations and services.
Ensuring Ethical and Responsible AI Use
As banks explore how to use AI in financial services, it’s crucial to prioritize ethical and responsible AI practices.
This includes ensuring that AI systems are transparent and explainable, particularly when they are used to make decisions that impact customers’ financial lives.
Banks must be vigilant about potential biases in AI models and take steps to mitigate these biases to ensure fair and equitable treatment of all customers.
Privacy and data protection should be at the forefront of AI implementation, with robust safeguards in place to protect sensitive customer information.
It’s important to establish clear guidelines and oversight mechanisms for the development and deployment of AI systems within the organization.
Banks should also engage with regulators and industry bodies to help shape responsible AI practices and standards for the financial services sector.
By prioritizing ethical and responsible AI use, banks can build trust with customers and stakeholders while mitigating potential risks associated with AI implementation.
Overcoming Challenges in AI Adoption
While the potential benefits of using AI in financial services are significant, banks face several challenges in adopting and scaling AI solutions.
One major hurdle is the integration of AI systems with legacy IT infrastructure, which can be complex and time-consuming.
Data quality and availability issues can also pose challenges, particularly when trying to train AI models on historical data that may be incomplete or inconsistent.
Regulatory uncertainty around the use of AI in certain areas of banking can create hesitation and slow down adoption.
There may also be resistance to change within the organization, requiring careful change management and employee training to ensure successful AI implementation.
Banks need to be prepared to invest significant resources in AI initiatives, recognizing that the benefits may take time to materialize.
By anticipating and addressing these challenges proactively, banks can increase their chances of success in leveraging AI to transform their operations and services.
Conclusion
As we’ve explored in this comprehensive guide, learning how to use AI in financial services is no longer optional for banks – it’s a necessity for staying competitive in an increasingly digital and data-driven industry.
From enhancing customer experiences and detecting fraud to improving risk assessment and streamlining compliance processes, AI offers a wide range of applications that can transform every aspect of banking.
By developing a strategic approach to AI implementation, building the necessary capabilities and infrastructure, and ensuring ethical and responsible use, banks can harness the full potential of AI to drive innovation, efficiency, and growth.
While the journey to becoming an AI-powered bank may be challenging, the potential rewards in terms of improved operations, enhanced customer satisfaction, and new business opportunities make it a worthwhile endeavor.
As AI technologies continue to evolve, banks that successfully integrate AI into their core operations will be well-positioned to thrive in the future of financial services.
The key to success lies in viewing AI not as a standalone technology, but as a fundamental component of a bank’s overall digital transformation strategy.
By embracing AI and continuously exploring new ways to leverage its capabilities, banks can create sustainable competitive advantages and deliver greater value to their customers in an increasingly complex and dynamic financial landscape.
Frequently Asked Questions
How is Gen AI used in financial services?
Generative AI (Gen AI) is being increasingly used in financial services in various ways:
- Customer Service: Gen AI powers chatbots and virtual assistants that can handle customer inquiries and provide personalized support.
- Content Generation: It can create personalized financial reports, investment summaries, and market analyses.
- Fraud Detection: Gen AI can generate synthetic data to train fraud detection models, improving their accuracy and robustness.
- Risk Assessment: It can analyze complex data patterns to generate more accurate risk profiles and credit scores.
- Product Recommendations: Gen AI can create personalized financial product recommendations based on individual customer data.
- Market Predictions: It can generate predictive models for market trends and investment opportunities.
- Regulatory Compliance: Gen AI can assist in generating and reviewing compliance reports and documentation.
- Process Automation: It can automate various financial processes by generating scripts and workflows.
Is there an AI tool for financial analysis?
Yes, there are several AI tools available for financial analysis:
- IBM Watson: Offers AI-powered financial analysis and risk management solutions.
- Alphasense: Uses AI for financial document search and analysis.
- Kensho: Provides AI-driven analytics for financial markets.
- Ayasdi: Offers AI-based financial crime detection and prevention.
- Numerai: Uses AI for stock market predictions and trading strategies.
- DataRobot: Provides automated machine learning for financial forecasting and risk assessment.
- Aidya: An AI-powered trading platform for financial markets.
- Kavout: Offers AI-driven investment research and portfolio management.
These tools leverage various AI technologies to analyze financial data, detect patterns, and provide insights for decision-making.
How to use AI as a financial analyst?
As a financial analyst, you can use AI in several ways to enhance your work:
- Data Processing: Use AI tools to quickly process and clean large datasets.
- Pattern Recognition: Leverage machine learning algorithms to identify trends and patterns in financial data.
- Predictive Analytics: Utilize AI models to forecast financial performance and market trends.
- Automated Reporting: Use AI-powered tools to generate financial reports and summaries.
- Sentiment Analysis: Employ NLP-based AI to analyze market sentiment from news and social media.
- Risk Assessment: Use AI models to assess and quantify various financial risks.
- Portfolio Optimization: Leverage AI algorithms to optimize investment portfolios based on various parameters.
- Fraud Detection: Implement AI-based systems to identify potential fraudulent activities.
To effectively use AI, familiarize yourself with popular AI tools and platforms, and consider taking courses in data science and machine learning to better understand and implement AI solutions in your work.
How to use AI in financial trading?
AI can be used in financial trading in several ways:
- Algorithmic Trading: Develop AI-powered trading algorithms that can execute trades based on pre-defined rules and market conditions.
- Market Analysis: Use machine learning models to analyze market trends and predict future price movements.
- Risk Management: Implement AI systems to assess and manage trading risks in real-time.
- High-Frequency Trading: Leverage AI for ultra-fast decision-making in high-frequency trading scenarios.
- Sentiment Analysis: Use NLP-based AI to analyze news, social media, and other sources to gauge market sentiment.
- Portfolio Management: Utilize AI algorithms to optimize and rebalance investment portfolios automatically.
- Anomaly Detection: Implement AI systems to detect unusual market behaviors or potential trading opportunities.
- Backtesting: Use AI to simulate and evaluate trading strategies using historical data.
To effectively use AI in financial trading, you’ll need to combine financial expertise with programming skills and knowledge of machine learning. It’s also crucial to stay updated on regulatory requirements and ethical considerations when implementing AI in trading systems.
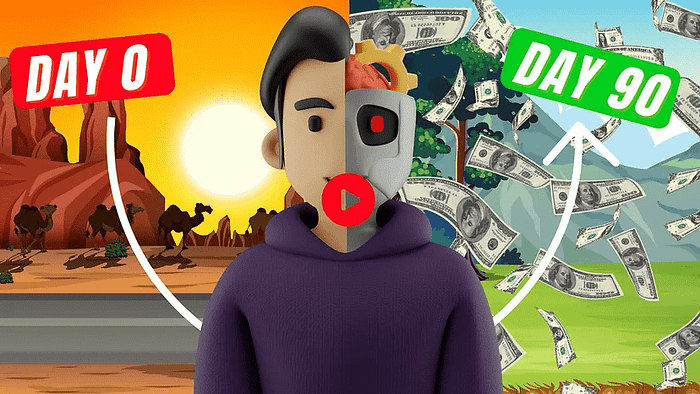
We strongly recommend that you check out our guide on how to take advantage of AI in today’s passive income economy.